LAPSE:2023.36583
Published Article
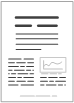
LAPSE:2023.36583
Application and Comparison of Machine Learning Methods for Mud Shale Petrographic Identification
August 3, 2023
Machine learning is the main technical means for lithofacies logging identification. As the main target of shale oil spatial distribution prediction, mud shale petrography is subjected to the constraints of stratigraphic inhomogeneity and logging information redundancy. Therefore, choosing the most applicable machine learning method for different geological characteristics and data situations is one of the key aspects of high-precision lithofacies identification. However, only a few studies have been conducted on the applicability of machine learning methods for mud shale petrography. This paper aims to identify lithofacies using commonly used machine learning methods. The study employs five supervised learning algorithms, namely Random Forest Algorithm (RF), BP Neural Network Algorithm (BPANN), Gradient Boosting Decision Tree Method (GBDT), Nearest Neighbor Method (KNN), and Vector Machine Method (SVM), as well as four unsupervised learning algorithms, namely K-means, DBSCAN, SOM, and MRGC. The results are evaluated using the confusion matrix, which provides the accuracy of each algorithm. The GBDT algorithm has better accuracy in supervised learning, while the K-means and DBSCAN algorithms have higher accuracy in unsupervised learning. Based on the comparison of different algorithms, it can be concluded that shale lithofacies identification poses challenges due to limited sample data and high overlapping degree of type distribution areas. Therefore, selecting the appropriate algorithm is crucial. Although supervised machine learning algorithms are generally accurate, they are limited by the data volume of lithofacies samples. Future research should focus on how to make the most of limited samples for supervised learning and combine unsupervised learning algorithms to explore lithofacies types of non-coring wells.
Record ID
Keywords
lithofacies classification, Machine Learning, shale
Subject
Suggested Citation
Liu R, Zhang L, Wang X, Zhang X, Liu X, He X, Zhao X, Xiao D, Cao Z. Application and Comparison of Machine Learning Methods for Mud Shale Petrographic Identification. (2023). LAPSE:2023.36583
Author Affiliations
Liu R: Institute of Unconventional Oil and Gas Development, Chongqing University of Science and Technology, Chongqing 401331, China [ORCID]
Zhang L: Institute of Unconventional Oil and Gas Development, Chongqing University of Science and Technology, Chongqing 401331, China; School of Petroleum Engineering, Chongqing University of Science and Technology, Chongqing 401331, China
Wang X: School of Earth Science, Northeast Petroleum University, Daqing 163318, China
Zhang X: School of Petroleum Engineering, Chongqing University of Science and Technology, Chongqing 401331, China
Liu X: Exploration and Development Research Institute of Liaohe Oilfield, Panjin 124010, China
He X: College of Petroleum Engineering, Xi’an Shiyou University, Xi’an 710065, China
Zhao X: College of Geosciences and Technology, Southwest Petroleum University, Chengdu 610500, China
Xiao D: Key Laboratory of Deep Oil and Gas, China University of Petroleum (East China), Qingdao 266580, China
Cao Z: School of Petroleum Engineering, Chongqing University of Science and Technology, Chongqing 401331, China [ORCID]
Zhang L: Institute of Unconventional Oil and Gas Development, Chongqing University of Science and Technology, Chongqing 401331, China; School of Petroleum Engineering, Chongqing University of Science and Technology, Chongqing 401331, China
Wang X: School of Earth Science, Northeast Petroleum University, Daqing 163318, China
Zhang X: School of Petroleum Engineering, Chongqing University of Science and Technology, Chongqing 401331, China
Liu X: Exploration and Development Research Institute of Liaohe Oilfield, Panjin 124010, China
He X: College of Petroleum Engineering, Xi’an Shiyou University, Xi’an 710065, China
Zhao X: College of Geosciences and Technology, Southwest Petroleum University, Chengdu 610500, China
Xiao D: Key Laboratory of Deep Oil and Gas, China University of Petroleum (East China), Qingdao 266580, China
Cao Z: School of Petroleum Engineering, Chongqing University of Science and Technology, Chongqing 401331, China [ORCID]
Journal Name
Processes
Volume
11
Issue
7
First Page
2042
Year
2023
Publication Date
2023-07-07
Published Version
ISSN
2227-9717
Version Comments
Original Submission
Other Meta
PII: pr11072042, Publication Type: Journal Article
Record Map
Published Article
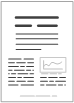
LAPSE:2023.36583
This Record
External Link
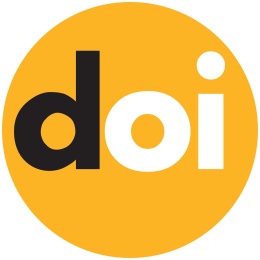
doi:10.3390/pr11072042
Publisher Version
Download
Meta
Record Statistics
Record Views
166
Version History
[v1] (Original Submission)
Aug 3, 2023
Verified by curator on
Aug 3, 2023
This Version Number
v1
Citations
Most Recent
This Version
URL Here
https://psecommunity.org/LAPSE:2023.36583
Original Submitter
Calvin Tsay
Links to Related Works