LAPSE:2023.3646
Published Article
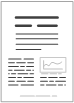
LAPSE:2023.3646
Energy Reduction with Super-Resolution Convolutional Neural Network for Ultrasound Tomography
February 22, 2023
This study addresses the issue of energy optimization by investigating solutions for the reduction of energy consumption in the diagnostics and monitoring of technological processes. The implementation of advanced process control is identified as a key approach for achieving energy savings and improving product quality, process efficiency, and production flexibility. The goal of this research is to develop a cost-effective system with a minimal number of ultrasound sensors, thus reducing the energy consumption of the overall system. To accomplish this, a novel method for obtaining high-resolution reconstruction in transmission ultrasound tomography (t-UST) is proposed. The method involves utilizing a convolutional neural network to take low-resolution measurements as input and output high-resolution sinograms that are used for tomography image reconstruction. This approach allows for the construction of a super-resolution sinogram by utilizing information hidden in the low-resolution measurement. The model is trained on simulation data and validated on real measurement data. The results of this technique demonstrate significant improvement compared to state-of-the-art methods. The study also highlights that UST measurements contain more information than previously thought, and this hidden information can be extracted and utilized with the use of machine learning techniques to further improve image quality and object recognition.
Record ID
Keywords
deep learning, energy consumption, energy optimization, Industry 4.0, inverse problems, Machine Learning, tomography
Suggested Citation
Wójcik D, Rymarczyk T, Przysucha B, Gołąbek M, Majerek D, Warowny T, Soleimani M. Energy Reduction with Super-Resolution Convolutional Neural Network for Ultrasound Tomography. (2023). LAPSE:2023.3646
Author Affiliations
Wójcik D: Faculty of Transport and Computer Science, WSEI University, 20-209 Lublin, Poland; Netrix S.A., Research & Development Centre, 20-704 Lublin, Poland [ORCID]
Rymarczyk T: Faculty of Transport and Computer Science, WSEI University, 20-209 Lublin, Poland; Netrix S.A., Research & Development Centre, 20-704 Lublin, Poland [ORCID]
Przysucha B: Faculty of Management, Lublin University of Technology, 20-618 Lublin, Poland [ORCID]
Gołąbek M: Netrix S.A., Research & Development Centre, 20-704 Lublin, Poland [ORCID]
Majerek D: Department of Applied Mathematics, Lublin University of Technology, 20-618 Lublin, Poland [ORCID]
Warowny T: Faculty of Management, Lublin University of Technology, 20-618 Lublin, Poland [ORCID]
Soleimani M: Department of Electronic and Electrical Engineering, University of Bath, Bath BA2 7AY, UK [ORCID]
Rymarczyk T: Faculty of Transport and Computer Science, WSEI University, 20-209 Lublin, Poland; Netrix S.A., Research & Development Centre, 20-704 Lublin, Poland [ORCID]
Przysucha B: Faculty of Management, Lublin University of Technology, 20-618 Lublin, Poland [ORCID]
Gołąbek M: Netrix S.A., Research & Development Centre, 20-704 Lublin, Poland [ORCID]
Majerek D: Department of Applied Mathematics, Lublin University of Technology, 20-618 Lublin, Poland [ORCID]
Warowny T: Faculty of Management, Lublin University of Technology, 20-618 Lublin, Poland [ORCID]
Soleimani M: Department of Electronic and Electrical Engineering, University of Bath, Bath BA2 7AY, UK [ORCID]
Journal Name
Energies
Volume
16
Issue
3
First Page
1387
Year
2023
Publication Date
2023-01-30
Published Version
ISSN
1996-1073
Version Comments
Original Submission
Other Meta
PII: en16031387, Publication Type: Journal Article
Record Map
Published Article
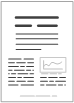
LAPSE:2023.3646
This Record
External Link
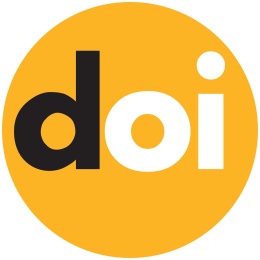
doi:10.3390/en16031387
Publisher Version
Download
Meta
Record Statistics
Record Views
78
Version History
[v1] (Original Submission)
Feb 22, 2023
Verified by curator on
Feb 22, 2023
This Version Number
v1
Citations
Most Recent
This Version
URL Here
https://psecommunity.org/LAPSE:2023.3646
Original Submitter
Auto Uploader for LAPSE
Links to Related Works