LAPSE:2023.36049
Published Article
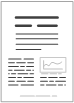
LAPSE:2023.36049
Study on the Optimal Double-Layer Electrode for a Non-Aqueous Vanadium-Iron Redox Flow Battery Using a Machine Learning Model Coupled with Genetic Algorithm
June 9, 2023
To boost the operational performance of a non-aqueous DES electrolyte-based vanadium-iron redox flow battery (RFB), our previous work proposed a double-layer porous electrode spliced by carbon paper and graphite felt. However, this electrode’s architecture still needs to be further optimized under different operational conditions. Hence, this paper proposes a multi-layer artificial neural network (ANN) model to predict the relationship between vanadium-iron RFB’s performance and double-layer electrode structural characteristics. A training dataset of ANN is generated by three-dimensional finite-element numerical simulations of the galvanostatic discharging process. In addition, a genetic algorithm (GA) is coupled to an ANN regression training process for optimizing the model parameters to elevate the accuracy of ANN prediction. The novelty of this work lies in this modified optimal method of a double-layer electrode for non-aqueous RFB driven by a machine learning (ML) model coupled with GA. The comparative result shows that the ML model reaches a satisfactory predictive accuracy, and the mean square error of this model is lower than other popular ML regression models. Based on the known region of operating conditions, the obtained results prove that this well-trained ML algorithm can be used to estimate whether a double-layer electrode should be applied to a non-aqueous vanadium-iron RFB and determine an appropriate thickness ratio for this double-layer electrode.
Record ID
Keywords
3D finite-element numerical simulation, artificial neural network, DES electrolyte, Genetic Algorithm, gradient porous electrode, Machine Learning, operational performance, redox flow battery, vanadium-iron
Suggested Citation
Ma Q, Fu W, Xu J, Wang Z, Xu Q. Study on the Optimal Double-Layer Electrode for a Non-Aqueous Vanadium-Iron Redox Flow Battery Using a Machine Learning Model Coupled with Genetic Algorithm. (2023). LAPSE:2023.36049
Author Affiliations
Ma Q: School of Mechanical and Vehicle Engineering, Nanchang Institute of Science and Technology, 998 Gezaoshan Road, Nanchang 330108, China; Institute for Energy Research, Jiangsu University, 301 Xuefu Road, Zhenjiang 212013, China [ORCID]
Fu W: Institute for Energy Research, Jiangsu University, 301 Xuefu Road, Zhenjiang 212013, China
Xu J: School of Advanced Manufacturing, Nanchang University, Nanchang 330031, China [ORCID]
Wang Z: School of Mechanical and Vehicle Engineering, Nanchang Institute of Science and Technology, 998 Gezaoshan Road, Nanchang 330108, China
Xu Q: Institute for Energy Research, Jiangsu University, 301 Xuefu Road, Zhenjiang 212013, China [ORCID]
Fu W: Institute for Energy Research, Jiangsu University, 301 Xuefu Road, Zhenjiang 212013, China
Xu J: School of Advanced Manufacturing, Nanchang University, Nanchang 330031, China [ORCID]
Wang Z: School of Mechanical and Vehicle Engineering, Nanchang Institute of Science and Technology, 998 Gezaoshan Road, Nanchang 330108, China
Xu Q: Institute for Energy Research, Jiangsu University, 301 Xuefu Road, Zhenjiang 212013, China [ORCID]
Journal Name
Processes
Volume
11
Issue
5
First Page
1529
Year
2023
Publication Date
2023-05-17
Published Version
ISSN
2227-9717
Version Comments
Original Submission
Other Meta
PII: pr11051529, Publication Type: Journal Article
Record Map
Published Article
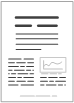
LAPSE:2023.36049
This Record
External Link
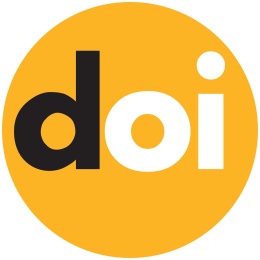
doi:10.3390/pr11051529
Publisher Version
Download
Meta
Record Statistics
Record Views
139
Version History
[v1] (Original Submission)
Jun 9, 2023
Verified by curator on
Jun 9, 2023
This Version Number
v1
Citations
Most Recent
This Version
URL Here
https://psecommunity.org/LAPSE:2023.36049
Original Submitter
Calvin Tsay
Links to Related Works