LAPSE:2023.35689
Published Article
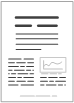
LAPSE:2023.35689
Predicting Energy Consumption in Residential Buildings Using Advanced Machine Learning Algorithms
May 23, 2023
The share of residential building energy consumption in global energy consumption has rapidly increased after the COVID-19 crisis. The accurate prediction of energy consumption under different indoor and outdoor conditions is an essential step towards improving energy efficiency and reducing carbon footprints in the residential building sector. In this paper, a PSO-optimized random forest classification algorithm is proposed to identify the most important factors contributing to residential heating energy consumption. A self-organizing map (SOM) approach is applied for feature dimensionality reduction, and an ensemble classification model based on the stacking method is trained on the dimensionality-reduced data. The results show that the stacking model outperforms the other models with an accuracy of 95.4% in energy consumption prediction. Finally, a causal inference method is introduced in addition to Shapley Additive Explanation (SHAP) to explore and analyze the factors influencing energy consumption. A clear causal relationship between water pipe temperature changes, air temperature, and building energy consumption is found, compensating for the neglect of temperature in the SHAP analysis. The findings of this research can help residential building owners/managers make more informed decisions around the selection of efficient heating management systems to save on energy bills.
Record ID
Keywords
energy consumption, Machine Learning, Net-Zero, prediction, residential building
Subject
Suggested Citation
Dinmohammadi F, Han Y, Shafiee M. Predicting Energy Consumption in Residential Buildings Using Advanced Machine Learning Algorithms. (2023). LAPSE:2023.35689
Author Affiliations
Dinmohammadi F: School of Computing and Engineering, University of West London, London W5 5RF, UK; The Bartlett Center of Advanced Spatial Analysis (CASA), University College London (UCL), Gower Street, London WC1E 6BTL, UK
Han Y: The Bartlett Center of Advanced Spatial Analysis (CASA), University College London (UCL), Gower Street, London WC1E 6BTL, UK
Shafiee M: School of Mechanical Engineering Sciences, University of Surrey, Guildford GU2 7XH, UK [ORCID]
Han Y: The Bartlett Center of Advanced Spatial Analysis (CASA), University College London (UCL), Gower Street, London WC1E 6BTL, UK
Shafiee M: School of Mechanical Engineering Sciences, University of Surrey, Guildford GU2 7XH, UK [ORCID]
Journal Name
Energies
Volume
16
Issue
9
First Page
3748
Year
2023
Publication Date
2023-04-27
Published Version
ISSN
1996-1073
Version Comments
Original Submission
Other Meta
PII: en16093748, Publication Type: Journal Article
Record Map
Published Article
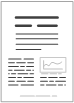
LAPSE:2023.35689
This Record
External Link
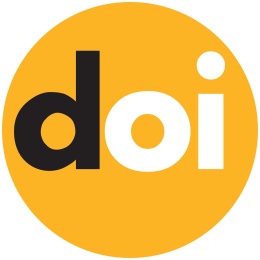
doi:10.3390/en16093748
Publisher Version
Download
Meta
Record Statistics
Record Views
139
Version History
[v1] (Original Submission)
May 23, 2023
Verified by curator on
May 23, 2023
This Version Number
v1
Citations
Most Recent
This Version
URL Here
https://psecommunity.org/LAPSE:2023.35689
Original Submitter
Calvin Tsay
Links to Related Works