LAPSE:2023.35574
Published Article
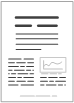
LAPSE:2023.35574
Research on Optimization of Profile Parameters in Screw Compressor Based on BP Neural Network and Genetic Algorithm
May 23, 2023
In order to accurately calculate the geometric characteristics of the twin-screw compressor and obtain the optimal profile parameters, a calculation method for the geometric characteristics of twin-screw compressors was proposed to simplify the profile parameter design in this paper. In this method, the database of geometric characteristics is established by back-propagation (BP) neural network, and the genetic algorithm is used to find the optimal profile design parameters. The effects of training methods and hidden layers on the calculation accuracy of neural network are discussed. The effects of profile parameters, including inner radius of the male rotor, protection angle, radius of the elliptic arc, outer radius of the female rotor on the comprehensive evaluation value composed of length of the contact line, blow hole area and area utilization rate, are analyzed. The results show that the time consumed for the database established by BP neural network is 92.8% shorter than that of the traditional method and the error is within 1.5% of the traditional method. Based on the genetic algorithm, compared with the original profile, the blow hole area of the screw compressor profile optimized by genetic algorithm is reduced by 54.8%, the length of contact line is increased by 1.57% and the area utilization rate is increased by 0.32%. The CFD numerical model is used to verify the optimization method, and it can be observed that the leakage through the blow hole of the optimized model is reduced, which makes the average mass flow rate increase by 5.2%, indicating the effectiveness of the rotor profile parameter optimization method.
Record ID
Keywords
BP neural network, Genetic Algorithm, geometric characteristics, screw compressor
Suggested Citation
Wang T, Qi Q, Zhang W, Zhan D. Research on Optimization of Profile Parameters in Screw Compressor Based on BP Neural Network and Genetic Algorithm. (2023). LAPSE:2023.35574
Author Affiliations
Wang T: School of Energy and Power Engineering, Zhengzhou University of Light Industry, Zhengzhou 450002, China
Qi Q: School of Energy and Power Engineering, Zhengzhou University of Light Industry, Zhengzhou 450002, China
Zhang W: School of Energy and Power Engineering, Zhengzhou University of Light Industry, Zhengzhou 450002, China; School of Energy and Power Engineering, Xi’an Jiaotong University, Xi’an 710049, China
Zhan D: School of Energy and Power Engineering, Zhengzhou University of Light Industry, Zhengzhou 450002, China
Qi Q: School of Energy and Power Engineering, Zhengzhou University of Light Industry, Zhengzhou 450002, China
Zhang W: School of Energy and Power Engineering, Zhengzhou University of Light Industry, Zhengzhou 450002, China; School of Energy and Power Engineering, Xi’an Jiaotong University, Xi’an 710049, China
Zhan D: School of Energy and Power Engineering, Zhengzhou University of Light Industry, Zhengzhou 450002, China
Journal Name
Energies
Volume
16
Issue
9
First Page
3632
Year
2023
Publication Date
2023-04-23
Published Version
ISSN
1996-1073
Version Comments
Original Submission
Other Meta
PII: en16093632, Publication Type: Journal Article
Record Map
Published Article
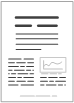
LAPSE:2023.35574
This Record
External Link
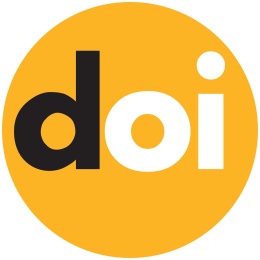
doi:10.3390/en16093632
Publisher Version
Download
Meta
Record Statistics
Record Views
130
Version History
[v1] (Original Submission)
May 23, 2023
Verified by curator on
May 23, 2023
This Version Number
v1
Citations
Most Recent
This Version
URL Here
https://psecommunity.org/LAPSE:2023.35574
Original Submitter
Calvin Tsay
Links to Related Works