LAPSE:2023.35468
Published Article
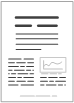
LAPSE:2023.35468
A Hybrid Algorithm-Level Ensemble Model for Imbalanced Credit Default Prediction in the Energy Industry
April 28, 2023
Credit default prediction for the energy industry is essential to promoting the healthy development of the energy industry in China. While previous studies have constructed various credit default prediction models with brilliant performance, the class-imbalance problem in the credit default dataset cannot be ignored, where the numbers of credit default cases are usually much smaller than the number of non-default ones. To address the class-imbalance problem, we proposed a novel CT-XGBoost model, which adds to XGBoost with two algorithm-level methods for class imbalance, including the cost-sensitive strategy and threshold method. Based on the credit default dataset consisting of energy corporates in western China, which suffers from the class-imbalance problem, the CT-XGBoost model achieves better performance than the conventional models. The results indicate that the proposed model can efficiently alleviate the inherent class-imbalance problem in the credit default dataset. Moreover, we analyze how the prediction performance is influenced by different parameter settings in the cost-sensitive strategy and threshold method. This study can help market investors and regulators precisely assess the credit risk in the energy industry and provides theoretical guidance to solving the class-imbalance problem in credit default prediction.
Record ID
Keywords
class imbalance, cost-sensitive, credit default prediction, energy industry, threshold method
Subject
Suggested Citation
Wang K, Wan J, Li G, Sun H. A Hybrid Algorithm-Level Ensemble Model for Imbalanced Credit Default Prediction in the Energy Industry. (2023). LAPSE:2023.35468
Author Affiliations
Wang K: School of Economics and Management, University of Chinese Academy of Sciences, Beijing 100190, China
Wan J: Institutes of Science and Development, Chinese Academy of Sciences, Beijing 100190, China; School of Public Policy and Management, University of Chinese Academy of Sciences, Beijing 100049, China
Li G: School of Business Administration, Northeastern University, Shenyang 110819, China [ORCID]
Sun H: Institutes of Science and Development, Chinese Academy of Sciences, Beijing 100190, China; School of Public Policy and Management, University of Chinese Academy of Sciences, Beijing 100049, China
Wan J: Institutes of Science and Development, Chinese Academy of Sciences, Beijing 100190, China; School of Public Policy and Management, University of Chinese Academy of Sciences, Beijing 100049, China
Li G: School of Business Administration, Northeastern University, Shenyang 110819, China [ORCID]
Sun H: Institutes of Science and Development, Chinese Academy of Sciences, Beijing 100190, China; School of Public Policy and Management, University of Chinese Academy of Sciences, Beijing 100049, China
Journal Name
Energies
Volume
15
Issue
14
First Page
5206
Year
2022
Publication Date
2022-07-18
Published Version
ISSN
1996-1073
Version Comments
Original Submission
Other Meta
PII: en15145206, Publication Type: Journal Article
Record Map
Published Article
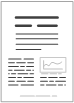
LAPSE:2023.35468
This Record
External Link
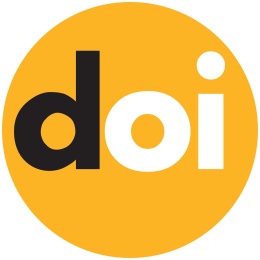
doi:10.3390/en15145206
Publisher Version
Download
Meta
Record Statistics
Record Views
154
Version History
[v1] (Original Submission)
Apr 28, 2023
Verified by curator on
Apr 28, 2023
This Version Number
v1
Citations
Most Recent
This Version
URL Here
https://psecommunity.org/LAPSE:2023.35468
Original Submitter
Auto Uploader for LAPSE
Links to Related Works