LAPSE:2023.34959
Published Article
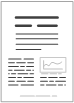
LAPSE:2023.34959
Multi-Horizon Wind Power Forecasting Using Multi-Modal Spatio-Temporal Neural Networks
April 28, 2023
Wind energy represents one of the leading renewable energy sectors and is considered instrumental in the ongoing decarbonization process. Accurate forecasts are essential for a reliable large-scale wind power integration, allowing efficient operation and maintenance, planning of unit commitment, and scheduling by system operators. However, due to non-stationarity, randomness, and intermittency, forecasting wind power is challenging. This work investigates a multi-modal approach for wind power forecasting by considering turbine-level time series collected from SCADA systems and high-resolution Numerical Weather Prediction maps. A neural architecture based on stacked Recurrent Neural Networks is proposed to process and combine different data sources containing spatio-temporal patterns. This architecture allows combining the local information from the turbine’s internal operating conditions with future meteorological data from the surrounding area. Specifically, this work focuses on multi-horizon turbine-level hourly forecasts for an entire wind farm with a lead time of 90 h. This work explores the impact of meteorological variables on different spatial scales, from full grids to cardinal point features, on wind power forecasts. Results show that a subset of features associated with all wind directions, even when spatially distant, can produce more accurate forecasts with respect to full grids and reduce computation times. The proposed model outperforms the linear regression baseline and the XGBoost regressor achieving an average skill score of 25%. Finally, the integration of SCADA data in the training process improved the predictions allowing the multi-modal neural network to model not only the meteorological patterns but also the turbine’s internal behavior.
Record ID
Keywords
multi-horizon forecasting, multi-modal neural network, wind power forecasting
Suggested Citation
Miele ES, Ludwig N, Corsini A. Multi-Horizon Wind Power Forecasting Using Multi-Modal Spatio-Temporal Neural Networks. (2023). LAPSE:2023.34959
Author Affiliations
Miele ES: Department of Mechanical and Aerospace Engineering, Sapienza University of Rome, 00184 Rome, Italy [ORCID]
Ludwig N: Cluster of Excellence−Machine Learning for Science, University of Tübingen, 72076 Tübingen, Germany [ORCID]
Corsini A: Department of Mechanical and Aerospace Engineering, Sapienza University of Rome, 00184 Rome, Italy [ORCID]
Ludwig N: Cluster of Excellence−Machine Learning for Science, University of Tübingen, 72076 Tübingen, Germany [ORCID]
Corsini A: Department of Mechanical and Aerospace Engineering, Sapienza University of Rome, 00184 Rome, Italy [ORCID]
Journal Name
Energies
Volume
16
Issue
8
First Page
3522
Year
2023
Publication Date
2023-04-18
Published Version
ISSN
1996-1073
Version Comments
Original Submission
Other Meta
PII: en16083522, Publication Type: Journal Article
Record Map
Published Article
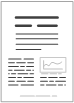
LAPSE:2023.34959
This Record
External Link
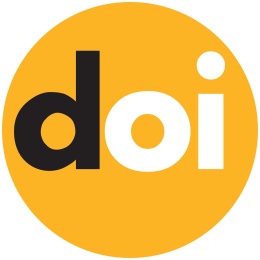
doi:10.3390/en16083522
Publisher Version
Download
Meta
Record Statistics
Record Views
81
Version History
[v1] (Original Submission)
Apr 28, 2023
Verified by curator on
Apr 28, 2023
This Version Number
v1
Citations
Most Recent
This Version
URL Here
https://psecommunity.org/LAPSE:2023.34959
Original Submitter
Auto Uploader for LAPSE
Links to Related Works