LAPSE:2023.34349
Published Article
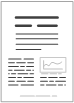
LAPSE:2023.34349
Time Series Clustering of Electricity Demand for Industrial Areas on Smart Grid
April 25, 2023
This study forecasts electricity demand in a smart grid environment. We present a prediction method that uses a combination of forecasting values based on time-series clustering. The clustering of normalized periodogram-based distances and autocorrelation-based distances are proposed as the time-series clustering methods. Trigonometrical transformation, Box−Cox transformation, autoregressive moving average (ARMA) errors, trend and seasonal components (TBATS), double seasonal Holt−Winters (DSHW), fractional autoregressive integrated moving average (FARIMA), ARIMA with regression (Reg-ARIMA), and neural network nonlinear autoregressive (NN-AR) are used for demand forecasting based on clustering. The results show that the time-series clustering method performs better than the method using the total amount of electricity demand in terms of the mean absolute percentage error (MAPE).
Record ID
Keywords
DSHW, NN-AR, smart grid, TBATS, time-series clustering
Subject
Suggested Citation
Son HG, Kim Y, Kim S. Time Series Clustering of Electricity Demand for Industrial Areas on Smart Grid. (2023). LAPSE:2023.34349
Author Affiliations
Son HG: Department of Short-term Demand Forecasting, Korea Power Exchange, Naju 58322, Korea
Kim Y: Department of Applied Statistics, Chung-ang University, Seoul 06974, Korea
Kim S: Department of Applied Statistics, Chung-ang University, Seoul 06974, Korea
Kim Y: Department of Applied Statistics, Chung-ang University, Seoul 06974, Korea
Kim S: Department of Applied Statistics, Chung-ang University, Seoul 06974, Korea
Journal Name
Energies
Volume
13
Issue
9
Article Number
E2377
Year
2020
Publication Date
2020-05-09
Published Version
ISSN
1996-1073
Version Comments
Original Submission
Other Meta
PII: en13092377, Publication Type: Journal Article
Record Map
Published Article
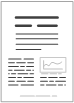
LAPSE:2023.34349
This Record
External Link
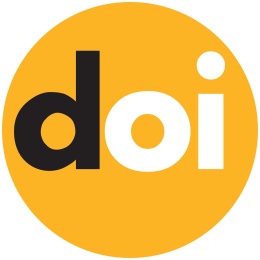
doi:10.3390/en13092377
Publisher Version
Download
Meta
Record Statistics
Record Views
105
Version History
[v1] (Original Submission)
Apr 25, 2023
Verified by curator on
Apr 25, 2023
This Version Number
v1
Citations
Most Recent
This Version
URL Here
https://psecommunity.org/LAPSE:2023.34349
Original Submitter
Auto Uploader for LAPSE
Links to Related Works