LAPSE:2023.34278
Published Article
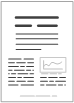
LAPSE:2023.34278
Electrical Energy Demand Forecasting Model Development and Evaluation with Maximum Overlap Discrete Wavelet Transform-Online Sequential Extreme Learning Machines Algorithms
April 25, 2023
To support regional electricity markets, accurate and reliable energy demand (G) forecast models are vital stratagems for stakeholders in this sector. An online sequential extreme learning machine (OS-ELM) model integrated with a maximum overlap discrete wavelet transform (MODWT) algorithm was developed using daily G data obtained from three regional campuses (i.e., Toowoomba, Ipswich, and Springfield) at the University of Southern Queensland, Australia. In training the objective and benchmark models, the partial autocorrelation function (PACF) was first employed to select the most significant lagged input variables that captured historical fluctuations in the G time-series data. To address the challenges of non-stationarities associated with the model development datasets, a MODWT technique was adopted to decompose the potential model inputs into their wavelet and scaling coefficients before executing the OS-ELM model. The MODWT-PACF-OS-ELM (MPOE) performance was tested and compared with the non-wavelet equivalent based on the PACF-OS-ELM (POE) model using a range of statistical metrics, including, but not limited to, the mean absolute percentage error (MAPE%). For all of the three datasets, a significantly greater accuracy was achieved with the MPOE model relative to the POE model resulting in an MAPE = 4.31% vs. MAPE = 11.31%, respectively, for the case of the Toowoomba dataset, and a similarly high performance for the other two campuses. Therefore, considering the high efficacy of the proposed methodology, the study claims that the OS-ELM model performance can be improved quite significantly by integrating the model with the MODWT algorithm.
Record ID
Keywords
energy security, MODWT, OS-ELM, predictive model for electricity demand, sustainable energy management systems, time-series forecasting, wavelet transformation
Subject
Suggested Citation
Al-Musaylh MS, Deo RC, Li Y. Electrical Energy Demand Forecasting Model Development and Evaluation with Maximum Overlap Discrete Wavelet Transform-Online Sequential Extreme Learning Machines Algorithms. (2023). LAPSE:2023.34278
Author Affiliations
Al-Musaylh MS: School of Sciences, Institute of Life Sciences and the Environment, University of Southern Queensland, Toowoomba, QLD 4350, Australia; Management Technical College, Southern Technical University, Basrah 61001, Iraq [ORCID]
Deo RC: School of Sciences, Institute of Life Sciences and the Environment, University of Southern Queensland, Toowoomba, QLD 4350, Australia [ORCID]
Li Y: School of Sciences, Institute of Life Sciences and the Environment, University of Southern Queensland, Toowoomba, QLD 4350, Australia [ORCID]
Deo RC: School of Sciences, Institute of Life Sciences and the Environment, University of Southern Queensland, Toowoomba, QLD 4350, Australia [ORCID]
Li Y: School of Sciences, Institute of Life Sciences and the Environment, University of Southern Queensland, Toowoomba, QLD 4350, Australia [ORCID]
Journal Name
Energies
Volume
13
Issue
9
Article Number
E2307
Year
2020
Publication Date
2020-05-06
Published Version
ISSN
1996-1073
Version Comments
Original Submission
Other Meta
PII: en13092307, Publication Type: Journal Article
Record Map
Published Article
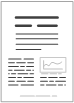
LAPSE:2023.34278
This Record
External Link
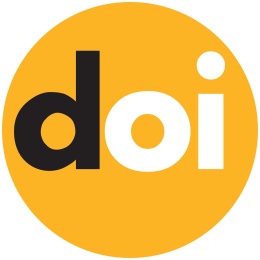
doi:10.3390/en13092307
Publisher Version
Download
Meta
Record Statistics
Record Views
81
Version History
[v1] (Original Submission)
Apr 25, 2023
Verified by curator on
Apr 25, 2023
This Version Number
v1
Citations
Most Recent
This Version
URL Here
https://psecommunity.org/LAPSE:2023.34278
Original Submitter
Auto Uploader for LAPSE
Links to Related Works