LAPSE:2023.34029
Published Article
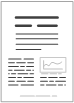
LAPSE:2023.34029
Traffic Noise Modelling Using Land Use Regression Model Based on Machine Learning, Statistical Regression and GIS
April 24, 2023
This study estimates the equivalent continuous sound pressure level (Leq) during peak daily periods (‘rush hour’) along the New Klang Valley Expressway (NKVE) in Shah Alam, Malaysia, using a land use regression (LUR) model based on machine learning, statistical regression, and geographical information systems (GIS). The research utilises two types of soft computing methods including machine learning (i.e., decision tree, random frost algorithms) and statistical regression (i.e., linear regression, support vector regression algorithms) to determine the best approach to create a prediction Leq map at the NKVE in Shah Alam, Malaysia. The selection of the best algorithm is accomplished by considering correlation, correlation coefficient, mean-absolute-error, mean-square-error, root-mean-square-error, and mean absolute percentage error. Traffic noise level was monitored using three sound level meters (TES 52A), and a traffic tally was done to analyse the traffic flow. Wind speed was gauged using a wind speed meter. The study relied on a variety of noise predictors including wind speed, digital elevation model, land use type (specifically, if it was residential, industrial, or natural reserve), residential density, road type (expressway, primary, and secondary) and traffic noise average (Leq). The above parameters were fed as inputs into the LUR model. Additional noise influencing factors such as traffic lights, intersections, road toll gates, gas stations, and public transportation infrastructures (bus stop and bus line) are also considered in this study. The models utilised parameters derived from LiDAR (Light Detection and Ranging) data, and various GIS (Geographical Information Systems) layers were extracted to produce the prediction maps. The results highlighted the superior performances by the machine learning (random forest) models compared to the statistical regression-based models.
Record ID
Keywords
GIS, land use regression model, LiDAR, Machine Learning, traffic noise modelling
Suggested Citation
Adulaimi AAA, Pradhan B, Chakraborty S, Alamri A. Traffic Noise Modelling Using Land Use Regression Model Based on Machine Learning, Statistical Regression and GIS. (2023). LAPSE:2023.34029
Author Affiliations
Adulaimi AAA: Centre for Advanced Modelling and Geospatial Information Systems (CAMGIS), Faculty of Engineering and Information Technology, University of Technology Sydney, Sydney, NSW 2007, Australia
Pradhan B: Centre for Advanced Modelling and Geospatial Information Systems (CAMGIS), Faculty of Engineering and Information Technology, University of Technology Sydney, Sydney, NSW 2007, Australia; Earth Observation Center, Institute of Climate Change, Universiti K [ORCID]
Chakraborty S: Centre for Advanced Modelling and Geospatial Information Systems (CAMGIS), Faculty of Engineering and Information Technology, University of Technology Sydney, Sydney, NSW 2007, Australia [ORCID]
Alamri A: Department of Geology and Geophysics, College of Science, King Saud University, Riyadh 11451, Saudi Arabia
Pradhan B: Centre for Advanced Modelling and Geospatial Information Systems (CAMGIS), Faculty of Engineering and Information Technology, University of Technology Sydney, Sydney, NSW 2007, Australia; Earth Observation Center, Institute of Climate Change, Universiti K [ORCID]
Chakraborty S: Centre for Advanced Modelling and Geospatial Information Systems (CAMGIS), Faculty of Engineering and Information Technology, University of Technology Sydney, Sydney, NSW 2007, Australia [ORCID]
Alamri A: Department of Geology and Geophysics, College of Science, King Saud University, Riyadh 11451, Saudi Arabia
Journal Name
Energies
Volume
14
Issue
16
First Page
5095
Year
2021
Publication Date
2021-08-18
Published Version
ISSN
1996-1073
Version Comments
Original Submission
Other Meta
PII: en14165095, Publication Type: Journal Article
Record Map
Published Article
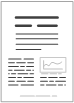
LAPSE:2023.34029
This Record
External Link
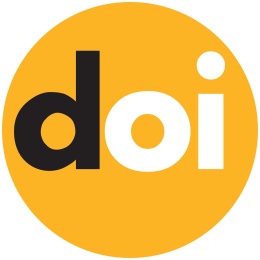
doi:10.3390/en14165095
Publisher Version
Download
Meta
Record Statistics
Record Views
115
Version History
[v1] (Original Submission)
Apr 24, 2023
Verified by curator on
Apr 24, 2023
This Version Number
v1
Citations
Most Recent
This Version
URL Here
https://psecommunity.org/LAPSE:2023.34029
Original Submitter
Auto Uploader for LAPSE
Links to Related Works