LAPSE:2023.33506
Published Article
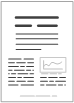
LAPSE:2023.33506
Machine Learning—A Review of Applications in Mineral Resource Estimation
April 21, 2023
Mineral resource estimation involves the determination of the grade and tonnage of a mineral deposit based on its geological characteristics using various estimation methods. Conventional estimation methods, such as geometric and geostatistical techniques, remain the most widely used methods for resource estimation. However, recent advances in computer algorithms have allowed researchers to explore the potential of machine learning techniques in mineral resource estimation. This study presents a comprehensive review of papers that have employed machine learning to estimate mineral resources. The review covers popular machine learning techniques and their implementation and limitations. Papers that performed a comparative analysis of both conventional and machine learning techniques were also considered. The literature shows that the machine learning models can accommodate several geological parameters and effectively approximate complex nonlinear relationships among them, exhibiting superior performance over the conventional techniques.
Record ID
Keywords
geostatistics, kriging, Machine Learning, ore, reserve estimation, resource estimation
Suggested Citation
Dumakor-Dupey NK, Arya S. Machine Learning—A Review of Applications in Mineral Resource Estimation. (2023). LAPSE:2023.33506
Author Affiliations
Dumakor-Dupey NK: Department of Mining and Mineral Engineering, College of Engineering and Mines, University of Alaska Fairbanks, Fairbanks, AK 99775, USA [ORCID]
Arya S: Department of Mining and Mineral Engineering, College of Engineering and Mines, University of Alaska Fairbanks, Fairbanks, AK 99775, USA [ORCID]
Arya S: Department of Mining and Mineral Engineering, College of Engineering and Mines, University of Alaska Fairbanks, Fairbanks, AK 99775, USA [ORCID]
Journal Name
Energies
Volume
14
Issue
14
First Page
4079
Year
2021
Publication Date
2021-07-06
Published Version
ISSN
1996-1073
Version Comments
Original Submission
Other Meta
PII: en14144079, Publication Type: Review
Record Map
Published Article
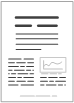
LAPSE:2023.33506
This Record
External Link
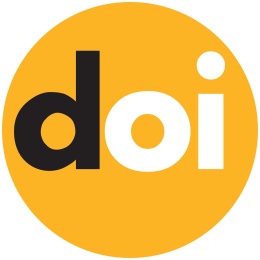
doi:10.3390/en14144079
Publisher Version
Download
Meta
Record Statistics
Record Views
89
Version History
[v1] (Original Submission)
Apr 21, 2023
Verified by curator on
Apr 21, 2023
This Version Number
v1
Citations
Most Recent
This Version
URL Here
https://psecommunity.org/LAPSE:2023.33506
Original Submitter
Auto Uploader for LAPSE
Links to Related Works