LAPSE:2023.33274
Published Article
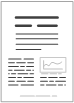
LAPSE:2023.33274
Adaptive Power Flow Prediction Based on Machine Learning
April 21, 2023
Power flow analysis is an inevitable methodology in the planning and operation of the power grid. It has been performed for the transmission system, however, along with the penetration of the distributed energy resources, the target has been expanded to the distribution system as well. However, it is not easy to apply the conventional method to the distribution system since the essential information for the power flow analysis, say the impedance and the topology, are not available for the distribution system. To this end, this paper proposes an alternative method based on practically available parameters at the terminal nodes without the precedent information. Since the available information is different between high-voltage and low-voltage systems, we develop two various machine learning schemes. Specifically, the high-voltage model incorporates the slack node voltage, which can be practically obtained at the substation, and yields a time-invariant model. On the other hand, the low voltage model utilizes the deviation of voltages at each node for the power changes, subsequently resulting in a time-varying model. The performance of the suggested models is also verified using numerical simulations. The results are analyzed and compared with another power flow scheme for the distribution system that the authors suggested beforehand.
Record ID
Keywords
distribution network, impedance estimation, Machine Learning, power flow, slack node voltage
Subject
Suggested Citation
Park J, Kodaira D, Agyeman KA, Jyung T, Han S. Adaptive Power Flow Prediction Based on Machine Learning. (2023). LAPSE:2023.33274
Author Affiliations
Park J: School of Electronic and Electrical Engineering, Kyungpook National University, Daegu 41566, Korea [ORCID]
Kodaira D: Department of Electrical Engineering, Tokyo University of Science, Tokyo 162-8601, Japan [ORCID]
Agyeman KA: School of Electronic and Electrical Engineering, Kyungpook National University, Daegu 41566, Korea
Jyung T: Korea Electric Power Corporation Engineering & Construction (KEPCO E&C), Gimcheon 39660, Korea
Han S: School of Electronic and Electrical Engineering, Kyungpook National University, Daegu 41566, Korea; Department of Electrical Engineering, Kyungpook National University, Daegu 41566, Korea
Kodaira D: Department of Electrical Engineering, Tokyo University of Science, Tokyo 162-8601, Japan [ORCID]
Agyeman KA: School of Electronic and Electrical Engineering, Kyungpook National University, Daegu 41566, Korea
Jyung T: Korea Electric Power Corporation Engineering & Construction (KEPCO E&C), Gimcheon 39660, Korea
Han S: School of Electronic and Electrical Engineering, Kyungpook National University, Daegu 41566, Korea; Department of Electrical Engineering, Kyungpook National University, Daegu 41566, Korea
Journal Name
Energies
Volume
14
Issue
13
First Page
3842
Year
2021
Publication Date
2021-06-25
Published Version
ISSN
1996-1073
Version Comments
Original Submission
Other Meta
PII: en14133842, Publication Type: Journal Article
Record Map
Published Article
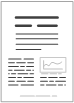
LAPSE:2023.33274
This Record
External Link
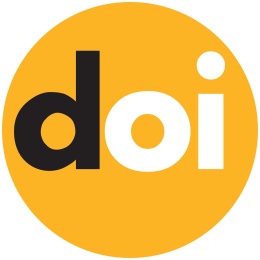
doi:10.3390/en14133842
Publisher Version
Download
Meta
Record Statistics
Record Views
78
Version History
[v1] (Original Submission)
Apr 21, 2023
Verified by curator on
Apr 21, 2023
This Version Number
v1
Citations
Most Recent
This Version
URL Here
https://psecommunity.org/LAPSE:2023.33274
Original Submitter
Auto Uploader for LAPSE
Links to Related Works