LAPSE:2023.33016
Published Article
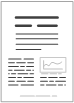
LAPSE:2023.33016
A Forecast-Based Load Management Approach for Commercial Buildings Demonstrated on an Integration of BEV
April 20, 2023
Load-forecasting problems have already been widely addressed with different approaches, granularities and objectives. Recent studies focused not only on deep learning methods but also on forecasting loads on single building level. This study aims to research problems and possibilities arising by using different load-forecasting techniques to manage loads. For that behavior of two neural networks, Long Short-Term Memory and Feed-Forward Neural Network as well as two statistical methods, standardized load profiles and personalized standardized load profiles are analyzed and assessed by using a sliding-window forecast approach. The results show that personalized standardized load profiles (MAE: 3.99) can perform similar to deep learning methods (for example, LSTM MAE: 4.47). However, because of the simplistic approach, load profiles are not able to adapt to new patterns. As a case study for evaluating the support of load-forecasting for applications in energy management systems, the integration of charging stations into an existing building is simulated by using load-forecasts to schedule the charging procedures. It is shown that forecast- based controlled charging can have a significant impact by lowering overload peaks exceeding the house connection point power limit (controlled charging 20.24 kW; uncontrolled charging: 65.15 kW) while slightly increasing average charging duration. It is concluded that integration of high flexible loads can be supported by using forecast-based energy management systems with regards to their limitations.
Record ID
Keywords
battery electric vehicles, charging strategies, load management, LSTM, Machine Learning, personalized standard load profiles, time-series prediction
Subject
Suggested Citation
Steens T, Telle JS, Hanke B, von Maydell K, Agert C, Di Modica GL, Engel B, Grottke M. A Forecast-Based Load Management Approach for Commercial Buildings Demonstrated on an Integration of BEV. (2023). LAPSE:2023.33016
Author Affiliations
Steens T: DLR Institute of Networked Energy Systems, Carl-von-Ossietzky-Str. 15, 26129 Oldenburg, Germany
Telle JS: DLR Institute of Networked Energy Systems, Carl-von-Ossietzky-Str. 15, 26129 Oldenburg, Germany [ORCID]
Hanke B: DLR Institute of Networked Energy Systems, Carl-von-Ossietzky-Str. 15, 26129 Oldenburg, Germany [ORCID]
von Maydell K: DLR Institute of Networked Energy Systems, Carl-von-Ossietzky-Str. 15, 26129 Oldenburg, Germany
Agert C: DLR Institute of Networked Energy Systems, Carl-von-Ossietzky-Str. 15, 26129 Oldenburg, Germany [ORCID]
Di Modica GL: Elenia Institute for High Voltage Technology and Power Systems, Technische Universität Braunschweig, Schleinitzstraße 23, 38106 Braunschweig, Germany
Engel B: Elenia Institute for High Voltage Technology and Power Systems, Technische Universität Braunschweig, Schleinitzstraße 23, 38106 Braunschweig, Germany
Grottke M: Hammer Real GmbH, Sylvensteinstr. 2, 81369 Munich, Germany
Telle JS: DLR Institute of Networked Energy Systems, Carl-von-Ossietzky-Str. 15, 26129 Oldenburg, Germany [ORCID]
Hanke B: DLR Institute of Networked Energy Systems, Carl-von-Ossietzky-Str. 15, 26129 Oldenburg, Germany [ORCID]
von Maydell K: DLR Institute of Networked Energy Systems, Carl-von-Ossietzky-Str. 15, 26129 Oldenburg, Germany
Agert C: DLR Institute of Networked Energy Systems, Carl-von-Ossietzky-Str. 15, 26129 Oldenburg, Germany [ORCID]
Di Modica GL: Elenia Institute for High Voltage Technology and Power Systems, Technische Universität Braunschweig, Schleinitzstraße 23, 38106 Braunschweig, Germany
Engel B: Elenia Institute for High Voltage Technology and Power Systems, Technische Universität Braunschweig, Schleinitzstraße 23, 38106 Braunschweig, Germany
Grottke M: Hammer Real GmbH, Sylvensteinstr. 2, 81369 Munich, Germany
Journal Name
Energies
Volume
14
Issue
12
First Page
3576
Year
2021
Publication Date
2021-06-16
Published Version
ISSN
1996-1073
Version Comments
Original Submission
Other Meta
PII: en14123576, Publication Type: Journal Article
Record Map
Published Article
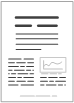
LAPSE:2023.33016
This Record
External Link
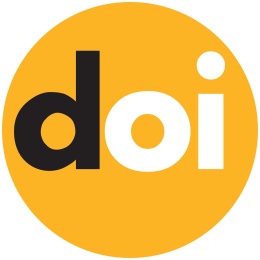
doi:10.3390/en14123576
Publisher Version
Download
Meta
Record Statistics
Record Views
68
Version History
[v1] (Original Submission)
Apr 20, 2023
Verified by curator on
Apr 20, 2023
This Version Number
v1
Citations
Most Recent
This Version
URL Here
https://psecommunity.org/LAPSE:2023.33016
Original Submitter
Auto Uploader for LAPSE
Links to Related Works