LAPSE:2023.32492
Published Article
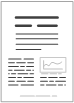
LAPSE:2023.32492
A Deep Learning Approach for Peak Load Forecasting: A Case Study on Panama
April 20, 2023
Predicting the future peak demand growth becomes increasingly important as more consumer loads and electric vehicles (EVs) start connecting to the grid. Accurate forecasts will enable energy suppliers to meet demand more reliably. However, this is a challenging problem since the peak demand is very nonlinear. This study addresses the research question of how deep learning methods, such as convolutional neural networks (CNNs) and long-short term memory (LSTM) can provide better support to these areas. The goal is to build a suitable forecasting model that can accurately predict the peak demand. Several data from 2004 to 2019 was collected from Panama’s power system to validate this study. Input features such as residential consumption and monthly economic index were considered for predicting peak demand. First, we introduced three different CNN architectures which were multivariate CNN, multivariate CNN-LSTM and multihead CNN. These were then benchmarked against LSTM. We found that the CNNs outperformed LSTM, with the multivariate CNN being the best performing model. To validate our initial findings, we then evaluated the robustness of the models against Gaussian noise. We demonstrated that CNNs were far more superior than LSTM and can support spatial-temporal time series data.
Record ID
Keywords
convolutional neural networks, deep learning, long-short term memory, peak load forecasting
Subject
Suggested Citation
Ibrahim B, Rabelo L. A Deep Learning Approach for Peak Load Forecasting: A Case Study on Panama. (2023). LAPSE:2023.32492
Author Affiliations
Ibrahim B: Industrial Engineering & Management Systems Department, University of Central Florida, Orlando, FL 32816, USA [ORCID]
Rabelo L: Industrial Engineering & Management Systems Department, University of Central Florida, Orlando, FL 32816, USA
Rabelo L: Industrial Engineering & Management Systems Department, University of Central Florida, Orlando, FL 32816, USA
Journal Name
Energies
Volume
14
Issue
11
First Page
3039
Year
2021
Publication Date
2021-05-24
Published Version
ISSN
1996-1073
Version Comments
Original Submission
Other Meta
PII: en14113039, Publication Type: Journal Article
Record Map
Published Article
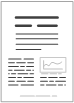
LAPSE:2023.32492
This Record
External Link
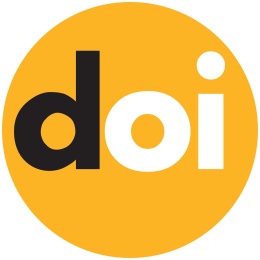
doi:10.3390/en14113039
Publisher Version
Download
Meta
Record Statistics
Record Views
74
Version History
[v1] (Original Submission)
Apr 20, 2023
Verified by curator on
Apr 20, 2023
This Version Number
v1
Citations
Most Recent
This Version
URL Here
https://psecommunity.org/LAPSE:2023.32492
Original Submitter
Auto Uploader for LAPSE
Links to Related Works