LAPSE:2023.32457
Published Article
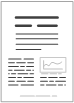
LAPSE:2023.32457
Crop Yield Estimation Using Deep Learning Based on Climate Big Data and Irrigation Scheduling
April 20, 2023
Deep learning has already been successfully used in the development of decision support systems in various domains. Therefore, there is an incentive to apply it in other important domains such as agriculture. Fertilizers, electricity, chemicals, human labor, and water are the components of total energy consumption in agriculture. Yield estimates are critical for food security, crop management, irrigation scheduling, and estimating labor requirements for harvesting and storage. Therefore, estimating product yield can reduce energy consumption. Two deep learning models, Long Short-Term Memory and Gated Recurrent Units, have been developed for the analysis of time-series data such as agricultural datasets. In this paper, the capabilities of these models and their extensions, called Bidirectional Long Short-Term Memory and Bidirectional Gated Recurrent Units, to predict end-of-season yields are investigated. The models use historical data, including climate data, irrigation scheduling, and soil water content, to estimate end-of-season yield. The application of this technique was tested for tomato and potato yields at a site in Portugal. The Bidirectional Long Short-Term memory outperformed the Gated Recurrent Units network, the Long Short-Term Memory, and the Bidirectional Gated Recurrent Units network on the validation dataset. The model was able to capture the nonlinear relationship between irrigation amount, climate data, and soil water content and predict yield with an MSE of 0.017 to 0.039. The performance of the Bidirectional Long Short-Term Memory in the test was compared with the most commonly used deep learning method, the Convolutional Neural Network, and machine learning methods including a Multi-Layer Perceptrons model and Random Forest Regression. The Bidirectional Long Short-Term Memory outperformed the other models with an R2 score between 0.97 and 0.99. The results show that analyzing agricultural data with the Long Short-Term Memory model improves the performance of the model in terms of accuracy. The Convolutional Neural Network model achieved the second-best performance. Therefore, the deep learning model has a remarkable ability to predict the yield at the end of the season.
Record ID
Keywords
agriculture, deep learning, irrigation management, LSTM, support decision-making algorithms, yield estimation
Subject
Suggested Citation
Alibabaei K, Gaspar PD, Lima TM. Crop Yield Estimation Using Deep Learning Based on Climate Big Data and Irrigation Scheduling. (2023). LAPSE:2023.32457
Author Affiliations
Alibabaei K: C-MAST Center for Mechanical and Aerospace Science and Technologies, University of Beira Interior, 6201-001 Covilhã, Portugal; Deparment of Electromechanical Engineering, University of Beira Interior, Rua Marquês d’Ávila e Bolama, 6201-001 Covilhã, [ORCID]
Gaspar PD: C-MAST Center for Mechanical and Aerospace Science and Technologies, University of Beira Interior, 6201-001 Covilhã, Portugal; Deparment of Electromechanical Engineering, University of Beira Interior, Rua Marquês d’Ávila e Bolama, 6201-001 Covilhã, [ORCID]
Lima TM: C-MAST Center for Mechanical and Aerospace Science and Technologies, University of Beira Interior, 6201-001 Covilhã, Portugal; Deparment of Electromechanical Engineering, University of Beira Interior, Rua Marquês d’Ávila e Bolama, 6201-001 Covilhã, [ORCID]
Gaspar PD: C-MAST Center for Mechanical and Aerospace Science and Technologies, University of Beira Interior, 6201-001 Covilhã, Portugal; Deparment of Electromechanical Engineering, University of Beira Interior, Rua Marquês d’Ávila e Bolama, 6201-001 Covilhã, [ORCID]
Lima TM: C-MAST Center for Mechanical and Aerospace Science and Technologies, University of Beira Interior, 6201-001 Covilhã, Portugal; Deparment of Electromechanical Engineering, University of Beira Interior, Rua Marquês d’Ávila e Bolama, 6201-001 Covilhã, [ORCID]
Journal Name
Energies
Volume
14
Issue
11
First Page
3004
Year
2021
Publication Date
2021-05-22
Published Version
ISSN
1996-1073
Version Comments
Original Submission
Other Meta
PII: en14113004, Publication Type: Journal Article
Record Map
Published Article
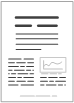
LAPSE:2023.32457
This Record
External Link
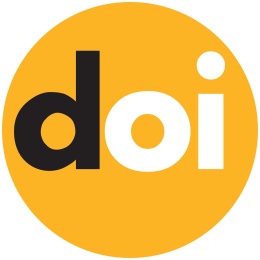
doi:10.3390/en14113004
Publisher Version
Download
Meta
Record Statistics
Record Views
72
Version History
[v1] (Original Submission)
Apr 20, 2023
Verified by curator on
Apr 20, 2023
This Version Number
v1
Citations
Most Recent
This Version
URL Here
https://psecommunity.org/LAPSE:2023.32457
Original Submitter
Auto Uploader for LAPSE
Links to Related Works