LAPSE:2023.32437
Published Article
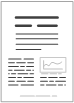
LAPSE:2023.32437
An Improved Particle Swarm Optimization with Chaotic Inertia Weight and Acceleration Coefficients for Optimal Extraction of PV Models Parameters
April 20, 2023
The efficiency of PV systems can be improved by accurate estimation of PV parameters. Parameter estimation of PV cells and modules is a challenging task as it requires accurate operation of PV cells and modules followed by an optimization tool that estimates their associated parameters. Mostly, population-based optimization tools are utilized for PV parameter estimation problems due to their computational intelligent behavior. However, most of them suffer from premature convergence problems, high computational burden, and often fall into local optimum solution. To mitigate these limitations, this paper presents an improved variant of particle swarm optimization (PSO) aiming to reduce shortcomings offered by conventional PSO for estimation of PV parameters. PSO is improved by introducing two strategies to control inertia weight and acceleration coefficients. At first, a sine chaotic inertia weight strategy is employed to attain an appropriate balance between local and global search. Afterward, a tangent chaotic strategy is utilized to guide acceleration coefficients in search of an optimal solution. The proposed algorithm is utilized to estimate the parameters of the PWP201 PV module, RTC France solar cell, and a JKM330P-72 PV module-based practical system. The obtained results indicate that the proposed technique avoids premature convergence and local optima stagnation of conventional PSO. Moreover, a comparison of obtained results with techniques available in the literature proves that the proposed methodology is an efficient, effective, and optimal tool to estimate PV modules and cells’ parameters.
Record ID
Keywords
acceleration coefficients, chaotic and tangent, inertia weight, parameter estimation, solar cell and modules
Subject
Suggested Citation
Kiani AT, Nadeem MF, Ahmed A, Khan IA, Alkhammash HI, Sajjad IA, Hussain B. An Improved Particle Swarm Optimization with Chaotic Inertia Weight and Acceleration Coefficients for Optimal Extraction of PV Models Parameters. (2023). LAPSE:2023.32437
Author Affiliations
Kiani AT: Department of Electrical Engineering, University of Engineering and Technology Taxila, Taxila 47080, Pakistan
Nadeem MF: Department of Electrical Engineering, University of Engineering and Technology Taxila, Taxila 47080, Pakistan; Clean and Resilient Energy Systems (CARES) Research Laboratory, Texas A&M University, Galveston, TX 77553, USA [ORCID]
Ahmed A: Department of Electrical Engineering, University of Engineering and Technology Taxila, Taxila 47080, Pakistan
Khan IA: Clean and Resilient Energy Systems (CARES) Research Laboratory, Texas A&M University, Galveston, TX 77553, USA [ORCID]
Alkhammash HI: Department of Electrical Engineering, College of Engineering, Taif University, P.O. Box 11099, Taif 21944, Saudi Arabia
Sajjad IA: Department of Electrical Engineering, University of Engineering and Technology Taxila, Taxila 47080, Pakistan [ORCID]
Hussain B: Department of Electrical Engineering, Pakistan Institute of Engineering and Applied Sciences (PIEAS), Islamabad 44000, Pakistan
Nadeem MF: Department of Electrical Engineering, University of Engineering and Technology Taxila, Taxila 47080, Pakistan; Clean and Resilient Energy Systems (CARES) Research Laboratory, Texas A&M University, Galveston, TX 77553, USA [ORCID]
Ahmed A: Department of Electrical Engineering, University of Engineering and Technology Taxila, Taxila 47080, Pakistan
Khan IA: Clean and Resilient Energy Systems (CARES) Research Laboratory, Texas A&M University, Galveston, TX 77553, USA [ORCID]
Alkhammash HI: Department of Electrical Engineering, College of Engineering, Taif University, P.O. Box 11099, Taif 21944, Saudi Arabia
Sajjad IA: Department of Electrical Engineering, University of Engineering and Technology Taxila, Taxila 47080, Pakistan [ORCID]
Hussain B: Department of Electrical Engineering, Pakistan Institute of Engineering and Applied Sciences (PIEAS), Islamabad 44000, Pakistan
Journal Name
Energies
Volume
14
Issue
11
First Page
2980
Year
2021
Publication Date
2021-05-21
Published Version
ISSN
1996-1073
Version Comments
Original Submission
Other Meta
PII: en14112980, Publication Type: Journal Article
Record Map
Published Article
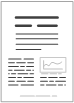
LAPSE:2023.32437
This Record
External Link
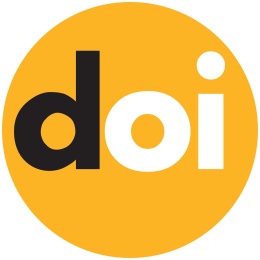
doi:10.3390/en14112980
Publisher Version
Download
Meta
Record Statistics
Record Views
58
Version History
[v1] (Original Submission)
Apr 20, 2023
Verified by curator on
Apr 20, 2023
This Version Number
v1
Citations
Most Recent
This Version
URL Here
https://psecommunity.org/LAPSE:2023.32437
Original Submitter
Auto Uploader for LAPSE
Links to Related Works