LAPSE:2023.32384
Published Article
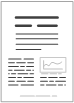
LAPSE:2023.32384
Temporal Patternization of Power Signatures for Appliance Classification in NILM
April 20, 2023
Non-Intrusive Load Monitoring (NILM) techniques are effective for managing energy and for addressing imbalances between the energy demand and supply. Various studies based on deep learning have reported the classification of appliances from aggregated power signals. In this paper, we propose a novel approach called a temporal bar graph, which patternizes the operational status of the appliances and time in order to extract the inherent features from the aggregated power signals for efficient load identification. To verify the effectiveness of the proposed method, a temporal bar graph was applied to the total power and tested on three state-of-the-art deep learning techniques that previously exhibited superior performance in image classification tasks—namely, Extreme Inception (Xception), Very Deep One Dimensional CNN (VDOCNN), and Concatenate-DenseNet121. The UK Domestic Appliance-Level Electricity (UK-DALE) and Tracebase datasets were used for our experiments. The results of the five-appliance case demonstrated that the accuracy and F1-score increased by 19.55% and 21.43%, respectively, on VDOCNN, and by 33.22% and 35.71%, respectively, on Xception. A performance comparison with the state-of-the-art deep learning methods and image-based spectrogram approach was conducted.
Record ID
Keywords
convolutional neural network (CNN), deep learning, load identification, non-intrusive load monitoring (NILM), temporal bar graph, temporal patternization
Subject
Suggested Citation
Kim H, Lim S. Temporal Patternization of Power Signatures for Appliance Classification in NILM. (2023). LAPSE:2023.32384
Author Affiliations
Journal Name
Energies
Volume
14
Issue
10
First Page
2931
Year
2021
Publication Date
2021-05-19
Published Version
ISSN
1996-1073
Version Comments
Original Submission
Other Meta
PII: en14102931, Publication Type: Journal Article
Record Map
Published Article
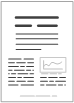
LAPSE:2023.32384
This Record
External Link
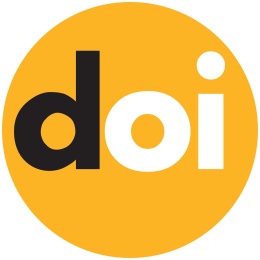
doi:10.3390/en14102931
Publisher Version
Download
Meta
Record Statistics
Record Views
87
Version History
[v1] (Original Submission)
Apr 20, 2023
Verified by curator on
Apr 20, 2023
This Version Number
v1
Citations
Most Recent
This Version
URL Here
https://psecommunity.org/LAPSE:2023.32384
Original Submitter
Auto Uploader for LAPSE
Links to Related Works