LAPSE:2023.32231
Published Article
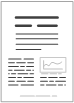
LAPSE:2023.32231
Comparison of Machine Learning Methods in Electrical Tomography for Detecting Moisture in Building Walls
April 20, 2023
This paper presents the results of research on the use of machine learning algorithms and electrical tomography in detecting humidity inside the walls of old buildings and structures. The object of research was a historical building in Wrocław, Poland, built in the first decade of the 19th century. Using the prototype of an electric tomograph of our own design, a number of voltage measurements were made on selected parts of the building. Many algorithmic methods have been preliminarily analyzed. Ultimately, the three models based on machine learning were selected: linear regression with SVM (support vector machine) learner, linear regression with least squares learner, and a multilayer perceptron neural network. The classical Gauss−Newton model was also used in the comparison. Both the experiments based on real measurements and simulation data showed a higher efficiency of machine learning methods than the Gauss−Newton method. The tomographic methods surpassed the point methods in measuring the dampness in the walls because they show a spatial image of the interior and not separate points of the examined cross-section. Research has shown that the selection of a machine learning model has a large impact on the quality of the results. Machine learning has a greater potential to create correct tomographic reconstructions than traditional mathematical methods. In this research, linear regression models performed slightly worse than neural networks.
Record ID
Keywords
dampness analysis, electrical tomography, linear regression, Machine Learning, moisture inspection, neural networks, nondestructive evaluation, SVM
Suggested Citation
Rymarczyk T, Kłosowski G, Hoła A, Sikora J, Wołowiec T, Tchórzewski P, Skowron S. Comparison of Machine Learning Methods in Electrical Tomography for Detecting Moisture in Building Walls. (2023). LAPSE:2023.32231
Author Affiliations
Rymarczyk T: Institute of Computer Science and Innovative Technologies, University of Economics and Innovation in Lublin, 20-209 Lublin, Poland; Research & Development Centre Netrix S.A., 20-704 Lublin, Poland [ORCID]
Kłosowski G: Faculty of Management, Lublin University of Technology, 20-618 Lublin, Poland [ORCID]
Hoła A: Faculty of Civil Engineering, Wrocław University of Science and Technology, 50-370 Wrocław, Poland [ORCID]
Sikora J: Institute of Computer Science and Innovative Technologies, University of Economics and Innovation in Lublin, 20-209 Lublin, Poland
Wołowiec T: Institute of Computer Science and Innovative Technologies, University of Economics and Innovation in Lublin, 20-209 Lublin, Poland
Tchórzewski P: Research & Development Centre Netrix S.A., 20-704 Lublin, Poland
Skowron S: Faculty of Management, Lublin University of Technology, 20-618 Lublin, Poland
Kłosowski G: Faculty of Management, Lublin University of Technology, 20-618 Lublin, Poland [ORCID]
Hoła A: Faculty of Civil Engineering, Wrocław University of Science and Technology, 50-370 Wrocław, Poland [ORCID]
Sikora J: Institute of Computer Science and Innovative Technologies, University of Economics and Innovation in Lublin, 20-209 Lublin, Poland
Wołowiec T: Institute of Computer Science and Innovative Technologies, University of Economics and Innovation in Lublin, 20-209 Lublin, Poland
Tchórzewski P: Research & Development Centre Netrix S.A., 20-704 Lublin, Poland
Skowron S: Faculty of Management, Lublin University of Technology, 20-618 Lublin, Poland
Journal Name
Energies
Volume
14
Issue
10
First Page
2777
Year
2021
Publication Date
2021-05-12
Published Version
ISSN
1996-1073
Version Comments
Original Submission
Other Meta
PII: en14102777, Publication Type: Journal Article
Record Map
Published Article
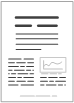
LAPSE:2023.32231
This Record
External Link
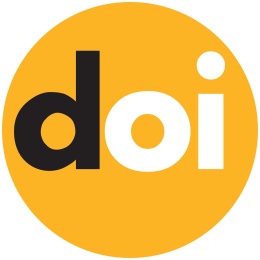
doi:10.3390/en14102777
Publisher Version
Download
Meta
Record Statistics
Record Views
87
Version History
[v1] (Original Submission)
Apr 20, 2023
Verified by curator on
Apr 20, 2023
This Version Number
v1
Citations
Most Recent
This Version
URL Here
https://psecommunity.org/LAPSE:2023.32231
Original Submitter
Auto Uploader for LAPSE
Links to Related Works