LAPSE:2023.32056
Published Article
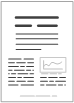
LAPSE:2023.32056
Rapid Model-Free State of Health Estimation for End-of-First-Life Electric Vehicle Batteries Using Impedance Spectroscopy
April 19, 2023
The continually expanding number of electric vehicles in circulation presents challenges in terms of end-of-life disposal, driving interest in the reuse of batteries for second-life applications. A key aspect of battery reuse is the quantification of the relative battery condition or state of health (SoH), to inform the subsequent battery application and to match batteries of similar capacity. Impedance spectroscopy has demonstrated potential for estimation of state of health, however, there is difficulty in interpreting results to estimate state of health reliably. This study proposes a model-free, convolutional-neural-network-based estimation scheme for the state of health of high-power lithium-ion batteries based on a dataset of impedance spectroscopy measurements from 13 end-of-first-life Nissan Leaf 2011 battery modules. As a baseline, this is compared with our previous approach, where parameters from a Randles equivalent circuit model (ECM) with and without dataset-specific adaptations to the ECM were extracted from the dataset to train a deep neural network refined using Bayesian hyperparameter optimisation. It is demonstrated that for a small dataset of 128 samples, the proposed method achieves good discrimination of high and low state of health batteries and superior prediction accuracy to the model-based approach by RMS error (1.974 SoH%) and peak error (4.935 SoH%) metrics without dataset-specific model adaptations to improve fit quality. This is accomplished while maintaining the competitive performance of the previous model-based approach when compared with previously proposed SoH estimation schemes.
Record ID
Keywords
battery second use, electric vehicles, lithium-ion batteries, Machine Learning, screening, state of health
Subject
Suggested Citation
Rastegarpanah A, Hathaway J, Stolkin R. Rapid Model-Free State of Health Estimation for End-of-First-Life Electric Vehicle Batteries Using Impedance Spectroscopy. (2023). LAPSE:2023.32056
Author Affiliations
Rastegarpanah A: Department of Metallurgy & Materials Science, University of Birmingham, Birmingham B15 2TT, UK; The Faraday Institution, Quad One, Harwell Science and Innovation Campus, Didcot OX11 0DG, UK [ORCID]
Hathaway J: Department of Metallurgy & Materials Science, University of Birmingham, Birmingham B15 2TT, UK
Stolkin R: Department of Metallurgy & Materials Science, University of Birmingham, Birmingham B15 2TT, UK; The Faraday Institution, Quad One, Harwell Science and Innovation Campus, Didcot OX11 0DG, UK
Hathaway J: Department of Metallurgy & Materials Science, University of Birmingham, Birmingham B15 2TT, UK
Stolkin R: Department of Metallurgy & Materials Science, University of Birmingham, Birmingham B15 2TT, UK; The Faraday Institution, Quad One, Harwell Science and Innovation Campus, Didcot OX11 0DG, UK
Journal Name
Energies
Volume
14
Issue
9
First Page
2597
Year
2021
Publication Date
2021-05-01
Published Version
ISSN
1996-1073
Version Comments
Original Submission
Other Meta
PII: en14092597, Publication Type: Journal Article
Record Map
Published Article
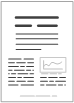
LAPSE:2023.32056
This Record
External Link
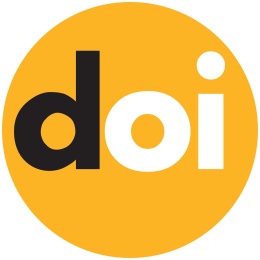
doi:10.3390/en14092597
Publisher Version
Download
Meta
Record Statistics
Record Views
64
Version History
[v1] (Original Submission)
Apr 19, 2023
Verified by curator on
Apr 19, 2023
This Version Number
v1
Citations
Most Recent
This Version
URL Here
https://psecommunity.org/LAPSE:2023.32056
Original Submitter
Auto Uploader for LAPSE
Links to Related Works