LAPSE:2023.31857
Published Article
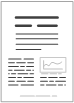
LAPSE:2023.31857
Review on Deep Neural Networks Applied to Low-Frequency NILM
April 19, 2023
This paper reviews non-intrusive load monitoring (NILM) approaches that employ deep neural networks to disaggregate appliances from low frequency data, i.e., data with sampling rates lower than the AC base frequency. The overall purpose of this review is, firstly, to gain an overview on the state of the research up to November 2020, and secondly, to identify worthwhile open research topics. Accordingly, we first review the many degrees of freedom of these approaches, what has already been done in the literature, and compile the main characteristics of the reviewed publications in an extensive overview table. The second part of the paper discusses selected aspects of the literature and corresponding research gaps. In particular, we do a performance comparison with respect to reported mean absolute error (MAE) and F1-scores and observe different recurring elements in the best performing approaches, namely data sampling intervals below 10 s, a large field of view, the usage of generative adversarial network (GAN) losses, multi-task learning, and post-processing. Subsequently, multiple input features, multi-task learning, and related research gaps are discussed, the need for comparative studies is highlighted, and finally, missing elements for a successful deployment of NILM approaches based on deep neural networks are pointed out. We conclude the review with an outlook on possible future scenarios.
Record ID
Keywords
deep learning, deep neural networks, load disaggregation, Machine Learning, NILM, non-intrusive load monitoring, review
Suggested Citation
Huber P, Calatroni A, Rumsch A, Paice A. Review on Deep Neural Networks Applied to Low-Frequency NILM. (2023). LAPSE:2023.31857
Author Affiliations
Huber P: iHomeLab, Engineering and Architecture, Lucerne University of Applied Sciences and Arts, 6048 Horw, Switzerland [ORCID]
Calatroni A: iHomeLab, Engineering and Architecture, Lucerne University of Applied Sciences and Arts, 6048 Horw, Switzerland [ORCID]
Rumsch A: iHomeLab, Engineering and Architecture, Lucerne University of Applied Sciences and Arts, 6048 Horw, Switzerland [ORCID]
Paice A: iHomeLab, Engineering and Architecture, Lucerne University of Applied Sciences and Arts, 6048 Horw, Switzerland [ORCID]
Calatroni A: iHomeLab, Engineering and Architecture, Lucerne University of Applied Sciences and Arts, 6048 Horw, Switzerland [ORCID]
Rumsch A: iHomeLab, Engineering and Architecture, Lucerne University of Applied Sciences and Arts, 6048 Horw, Switzerland [ORCID]
Paice A: iHomeLab, Engineering and Architecture, Lucerne University of Applied Sciences and Arts, 6048 Horw, Switzerland [ORCID]
Journal Name
Energies
Volume
14
Issue
9
First Page
2390
Year
2021
Publication Date
2021-04-23
Published Version
ISSN
1996-1073
Version Comments
Original Submission
Other Meta
PII: en14092390, Publication Type: Review
Record Map
Published Article
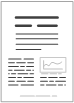
LAPSE:2023.31857
This Record
External Link
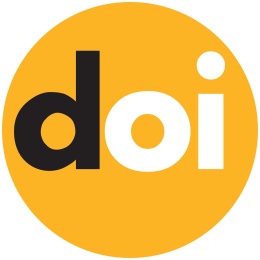
doi:10.3390/en14092390
Publisher Version
Download
Meta
Record Statistics
Record Views
67
Version History
[v1] (Original Submission)
Apr 19, 2023
Verified by curator on
Apr 19, 2023
This Version Number
v1
Citations
Most Recent
This Version
URL Here
https://psecommunity.org/LAPSE:2023.31857
Original Submitter
Auto Uploader for LAPSE
Links to Related Works