LAPSE:2023.31716
Published Article
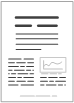
LAPSE:2023.31716
Battery State of Health Estimation with Improved Generalization Using Parallel Layer Extreme Learning Machine
April 19, 2023
The online estimation of battery state of health (SOH) is crucial to ensure the reliability of the energy supply in electric and hybrid vehicles. An approach for enhancing the generalization of SOH estimation using a parallel layer extreme learning machine (PL-ELM) algorithm is analyzed in this paper. The deterministic and stable PL-ELM model is designed to overcome the drift problem that is associated with some conventional machine learning algorithms; hence, extending the application of a single SOH estimation model over a large set of batteries of the same type. The PL-ELM model was trained with selected features that characterize the SOH. These features are acquired as the discrete variation of indicator variables including voltage, state of charge (SOC), and energy releasable by the battery. The model training was performed with an experimental battery dataset collected at room temperature under a constant current load condition at discharge phases. Model validation was performed with a dataset of other batteries of the same type that were aged under a constant load condition. An optimum performance with low error variance was obtained from the model result. The root mean square error (RMSE) of the validated model varies from 0.064% to 0.473%, and the mean absolute error (MAE) error from 0.034% to 0.355% for the battery sets tested. On the basis of performance, the model was compared with a deterministic extreme learning machine (ELM) and an incremental capacity analysis (ICA)-based scheme from the literature. The algorithm was tested on a Texas F28379D microcontroller unit (MCU) board with an average execution speed of 93 μs in real time, and 0.9305% CPU occupation. These results suggest that the model is suitable for online applications.
Record ID
Keywords
Artificial Intelligence, automotive, Batteries, electric vehicles, energy reliability, hybrid vehicles, improved generalization, online application, parallel layer extreme learning machine, state of health
Subject
Suggested Citation
Ezemobi E, Tonoli A, Silvagni M. Battery State of Health Estimation with Improved Generalization Using Parallel Layer Extreme Learning Machine. (2023). LAPSE:2023.31716
Author Affiliations
Ezemobi E: Department of Mechanical and Aerospace Engineering, Politecnico di Torino, 10129 Torino, Italy [ORCID]
Tonoli A: Department of Mechanical and Aerospace Engineering, Politecnico di Torino, 10129 Torino, Italy [ORCID]
Silvagni M: Department of Mechanical and Aerospace Engineering, Politecnico di Torino, 10129 Torino, Italy [ORCID]
Tonoli A: Department of Mechanical and Aerospace Engineering, Politecnico di Torino, 10129 Torino, Italy [ORCID]
Silvagni M: Department of Mechanical and Aerospace Engineering, Politecnico di Torino, 10129 Torino, Italy [ORCID]
Journal Name
Energies
Volume
14
Issue
8
First Page
2243
Year
2021
Publication Date
2021-04-16
Published Version
ISSN
1996-1073
Version Comments
Original Submission
Other Meta
PII: en14082243, Publication Type: Journal Article
Record Map
Published Article
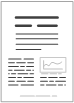
LAPSE:2023.31716
This Record
External Link
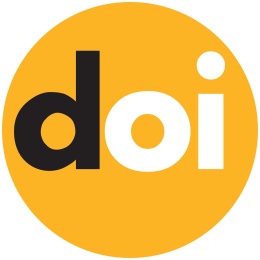
doi:10.3390/en14082243
Publisher Version
Download
Meta
Record Statistics
Record Views
133
Version History
[v1] (Original Submission)
Apr 19, 2023
Verified by curator on
Apr 19, 2023
This Version Number
v1
Citations
Most Recent
This Version
URL Here
https://psecommunity.org/LAPSE:2023.31716
Original Submitter
Auto Uploader for LAPSE
Links to Related Works