LAPSE:2023.31458
Published Article
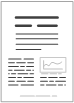
LAPSE:2023.31458
Research on Data-Driven Optimal Scheduling of Power System
April 18, 2023
The uncertainty of output makes it difficult to effectively solve the economic security dispatching problem of the power grid when a high proportion of renewable energy generating units are integrated into the power grid. Based on the proximal policy optimization (PPO) algorithm, a safe and economical grid scheduling method is designed. First, constraints on the safe and economical operation of renewable energy power systems are defined. Then, the quintuple of Markov decision process is defined under the framework of deep reinforcement learning, and the dispatching optimization problem is transformed into Markov decision process. To solve the problem of low sample data utilization in online reinforcement learning strategies, a PPO optimization algorithm based on the Kullback−Leibler (KL) divergence penalty factor and importance sampling technique is proposed, which transforms on-policy into off-policy and improves sample utilization. Finally, the simulation analysis of the example shows that in a power system with a high proportion of renewable energy generating units connected to the grid, the proposed scheduling strategy can meet the load demand under different load trends. In the dispatch cycle with different renewable energy generation rates, renewable energy can be absorbed to the maximum extent to ensure the safe and economic operation of the grid.
Record ID
Keywords
deep reinforcement learning, grid dispatching optimization, importance sampling, proximal policy optimization algorithm
Subject
Suggested Citation
Luo J, Zhang W, Wang H, Wei W, He J. Research on Data-Driven Optimal Scheduling of Power System. (2023). LAPSE:2023.31458
Author Affiliations
Luo J: School of Information and Automation, Qilu University of Technology (Shandong Academy of Sciences), Jinan 250353, China
Zhang W: School of Information and Automation, Qilu University of Technology (Shandong Academy of Sciences), Jinan 250353, China
Wang H: Department of Electrical Engineering, Shandong University, Jinan 250061, China
Wei W: Automation Academy, Huazhong University of Science and Technology, Wuhan 430074, China
He J: School of Information and Automation, Qilu University of Technology (Shandong Academy of Sciences), Jinan 250353, China
Zhang W: School of Information and Automation, Qilu University of Technology (Shandong Academy of Sciences), Jinan 250353, China
Wang H: Department of Electrical Engineering, Shandong University, Jinan 250061, China
Wei W: Automation Academy, Huazhong University of Science and Technology, Wuhan 430074, China
He J: School of Information and Automation, Qilu University of Technology (Shandong Academy of Sciences), Jinan 250353, China
Journal Name
Energies
Volume
16
Issue
6
First Page
2926
Year
2023
Publication Date
2023-03-22
Published Version
ISSN
1996-1073
Version Comments
Original Submission
Other Meta
PII: en16062926, Publication Type: Journal Article
Record Map
Published Article
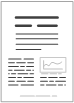
LAPSE:2023.31458
This Record
External Link
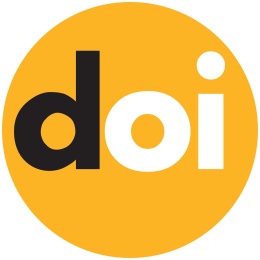
doi:10.3390/en16062926
Publisher Version
Download
Meta
Record Statistics
Record Views
61
Version History
[v1] (Original Submission)
Apr 18, 2023
Verified by curator on
Apr 18, 2023
This Version Number
v1
Citations
Most Recent
This Version
URL Here
https://psecommunity.org/LAPSE:2023.31458
Original Submitter
Auto Uploader for LAPSE
Links to Related Works