LAPSE:2023.31106
Published Article
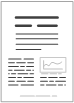
LAPSE:2023.31106
Electric Vehicle Battery-Connected Parallel Distribution Generators for Intelligent Demand Management in Smart Microgrids
April 18, 2023
Renewable energy penetration increases Smart Grid (SG) instability. A power balance between consumption and production can mitigate this instability. For this, intelligent and optimizing techniques can be used to properly combine and manage storage devices like Electric Vehicle Batteries (EVBs) with Demand-Side Management (DSM) strategies. The EVB helps distribution networks with auxiliary services, backup power, reliability, demand response, peak shaving, lower renewable power production’s climate unpredictability, etc. In this paper, a new energy management system based on Artificial Neural Networks (ANNs) is developed to maximize the performance of islanded SG-connected EVBs. The proposed ANN controller can operate at specified periods based on the demand curve and EVB charge level to implement a peak load shaving (PLS) DSM strategy. The intelligent controller’s inputs include the time of day and the EVB’s State of Charge (SOC). After the controller detects a peak demand, it alerts the EVB to start delivering power. This decrease in peak demand enhances the load factor and benefits both SG investors and end users. In this study, the adopted SG includes five parallel Distribution Generators (DGs) powered by renewable resources, which are three solar Photovoltaics (PVs) and two Wind Turbines (WTs). Sharing power among these DGs ensures the SG’s stability and efficiency. To fulfill demand problem-free, this study dynamically alters the power flow toward equity in power sharing using virtual impedance-based adaptive primary control level. This study proposes a decentralized robust hierarchical secondary control system employing Genetic Algorithm (GA)-optimized Proportional-Integral (PI) controller parameters with fine-grained online tuning using ANNs to restore frequency and voltage deviations. The proposed system is evidenced to be effective through MATLAB simulations and real-time data analysis on the ThingSpeak platform using internet energy technology. Our presented model not only benefits users by enhancing their utility but also reduces energy costs with robust implementation of a control structure by restoring any frequency and voltage deviations by distributing power equally among DGs regardless of demand condition variations.
Record ID
Keywords
artificial neural network, distribution generators, Genetic Algorithm, microgrid, power sharing, secondary control, virtual impedance
Suggested Citation
Jasim AM, Jasim BH, Neagu BC, Attila S. Electric Vehicle Battery-Connected Parallel Distribution Generators for Intelligent Demand Management in Smart Microgrids. (2023). LAPSE:2023.31106
Author Affiliations
Jasim AM: Electrical Engineering Department, University of Basrah, Basrah 61001, Iraq; Department of Communications Engineering, Iraq University College, Basrah 61001, Iraq [ORCID]
Jasim BH: Electrical Engineering Department, University of Basrah, Basrah 61001, Iraq [ORCID]
Neagu BC: Power Engineering Department, Gheorghe Asachi Technical University of Iasi, 700050 Iasi, Romania [ORCID]
Attila S: Power Systems Department, Politehnica University Timisoara, No. 2, V. Parvan Bvd., 300223 Timisoara, Romania [ORCID]
Jasim BH: Electrical Engineering Department, University of Basrah, Basrah 61001, Iraq [ORCID]
Neagu BC: Power Engineering Department, Gheorghe Asachi Technical University of Iasi, 700050 Iasi, Romania [ORCID]
Attila S: Power Systems Department, Politehnica University Timisoara, No. 2, V. Parvan Bvd., 300223 Timisoara, Romania [ORCID]
Journal Name
Energies
Volume
16
Issue
6
First Page
2570
Year
2023
Publication Date
2023-03-08
Published Version
ISSN
1996-1073
Version Comments
Original Submission
Other Meta
PII: en16062570, Publication Type: Journal Article
Record Map
Published Article
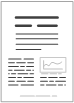
LAPSE:2023.31106
This Record
External Link
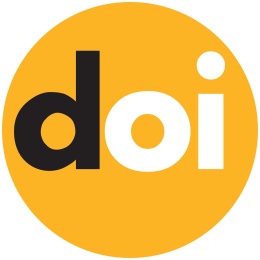
doi:10.3390/en16062570
Publisher Version
Download
Meta
Record Statistics
Record Views
102
Version History
[v1] (Original Submission)
Apr 18, 2023
Verified by curator on
Apr 18, 2023
This Version Number
v1
Citations
Most Recent
This Version
URL Here
https://psecommunity.org/LAPSE:2023.31106
Original Submitter
Auto Uploader for LAPSE
Links to Related Works