LAPSE:2023.30498
Published Article
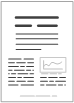
LAPSE:2023.30498
A Data-Mining Approach for Wind Turbine Fault Detection Based on SCADA Data Analysis Using Artificial Neural Networks
April 14, 2023
Wind energy has shown significant growth in terms of installed power in the last decade. However, one of the most critical problems for a wind farm is represented by Operation and Maintenance (O&M) costs, which can represent 20−30% of the total costs related to power generation. Various monitoring methodologies targeted to the identification of faults, such as vibration analysis or analysis of oils, are often used. However, they have the main disadvantage of involving additional costs as they usually entail the installation of other sensors to provide real-time control of the system. In this paper, we propose a methodology based on machine learning techniques using data from SCADA systems (Supervisory Control and Data Acquisition). Since these systems are generally already implemented on most wind turbines, they provide a large amount of data without requiring extra sensors. In particular, we developed models using Artificial Neural Networks (ANN) to characterize the behavior of some of the main components of the wind turbine, such as gearbox and generator, and predict operating anomalies. The proposed method is tested on real wind turbines in Italy to verify its effectiveness and applicability, and it was demonstrated to be able to provide significant help for the maintenance of a wind farm.
Record ID
Keywords
artificial neural networks, condition monitoring, Fault Detection, gearbox, generator, predictive maintenance, wind turbine
Suggested Citation
Santolamazza A, Dadi D, Introna V. A Data-Mining Approach for Wind Turbine Fault Detection Based on SCADA Data Analysis Using Artificial Neural Networks. (2023). LAPSE:2023.30498
Author Affiliations
Journal Name
Energies
Volume
14
Issue
7
First Page
1845
Year
2021
Publication Date
2021-03-26
Published Version
ISSN
1996-1073
Version Comments
Original Submission
Other Meta
PII: en14071845, Publication Type: Journal Article
Record Map
Published Article
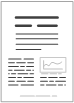
LAPSE:2023.30498
This Record
External Link
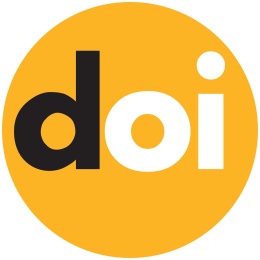
doi:10.3390/en14071845
Publisher Version
Download
Meta
Record Statistics
Record Views
120
Version History
[v1] (Original Submission)
Apr 14, 2023
Verified by curator on
Apr 14, 2023
This Version Number
v1
Citations
Most Recent
This Version
URL Here
https://psecommunity.org/LAPSE:2023.30498
Original Submitter
Auto Uploader for LAPSE
Links to Related Works