LAPSE:2023.29788
Published Article
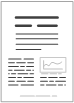
LAPSE:2023.29788
Machine Learning-Based Predictive Modelling of Biodiesel Production—A Comparative Perspective
April 13, 2023
Owing to the ever-growing impetus towards the development of eco-friendly and low carbon footprint energy solutions, biodiesel production and usage have been the subject of tremendous research efforts. The biodiesel production process is driven by several process parameters, which must be maintained at optimum levels to ensure high productivity. Since biodiesel productivity and quality are also dependent on the various raw materials involved in transesterification, physical experiments are necessary to make any estimation regarding them. However, a brute force approach of carrying out physical experiments until the optimal process parameters have been achieved will not succeed, due to a large number of process parameters and the underlying non-linear relation between the process parameters and responses. In this regard, a machine learning-based prediction approach is used in this paper to quantify the response features of the biodiesel production process as a function of the process parameters. Three powerful machine learning algorithms—linear regression, random forest regression and AdaBoost regression are comprehensively studied in this work. Furthermore, two separate examples—one involving biodiesel yield, the other regarding biodiesel free fatty acid conversion percentage—are illustrated. It is seen that both random forest regression and AdaBoost regression can achieve high accuracy in predictive modelling of biodiesel yield and free fatty acid conversion percentage. However, AdaBoost may be a more suitable approach for biodiesel production modelling, as it achieves the best accuracy amongst the tested algorithms. Moreover, AdaBoost can be more quickly deployed, as it was seen to be insensitive to number of regressors used.
Record ID
Keywords
AdaBoost regression, biodiesel, linear regression, Machine Learning, random forest regression
Subject
Suggested Citation
Gupta KK, Kalita K, Ghadai RK, Ramachandran M, Gao XZ. Machine Learning-Based Predictive Modelling of Biodiesel Production—A Comparative Perspective. (2023). LAPSE:2023.29788
Author Affiliations
Gupta KK: Department of Mechanical Engineering, MPSTME, SVKM’s Narsee Monjee Institute of Management Studies (NMIMS), Shirpur Campus, Dhule 425 405, India
Kalita K: Department of Mechanical Engineering, Vel Tech Rangarajan Dr. Sagunthala R&D Institute of Science and Technology, Avadi 600 062, India
Ghadai RK: Department of Mechanical Engineering, Sikkim Manipal Institute of Technology, Sikkim Manipal University, Majitar 737 136, India
Ramachandran M: Data Analytics Lab, REST Labs, Kaveripattinam, Krishnagiri 635 112, India
Gao XZ: School of Computing, University of Eastern Finland, FI-70211 Kuopio, Finland
Kalita K: Department of Mechanical Engineering, Vel Tech Rangarajan Dr. Sagunthala R&D Institute of Science and Technology, Avadi 600 062, India
Ghadai RK: Department of Mechanical Engineering, Sikkim Manipal Institute of Technology, Sikkim Manipal University, Majitar 737 136, India
Ramachandran M: Data Analytics Lab, REST Labs, Kaveripattinam, Krishnagiri 635 112, India
Gao XZ: School of Computing, University of Eastern Finland, FI-70211 Kuopio, Finland
Journal Name
Energies
Volume
14
Issue
4
First Page
1122
Year
2021
Publication Date
2021-02-20
Published Version
ISSN
1996-1073
Version Comments
Original Submission
Other Meta
PII: en14041122, Publication Type: Journal Article
Record Map
Published Article
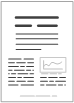
LAPSE:2023.29788
This Record
External Link
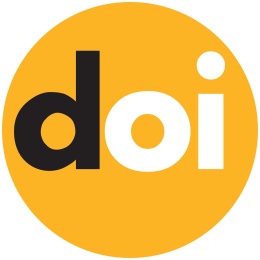
doi:10.3390/en14041122
Publisher Version
Download
Meta
Record Statistics
Record Views
62
Version History
[v1] (Original Submission)
Apr 13, 2023
Verified by curator on
Apr 13, 2023
This Version Number
v1
Citations
Most Recent
This Version
URL Here
https://psecommunity.org/LAPSE:2023.29788
Original Submitter
Auto Uploader for LAPSE
Links to Related Works