LAPSE:2023.26489
Published Article
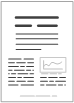
LAPSE:2023.26489
State-of-Health Prediction for Lithium-Ion Batteries Based on a Novel Hybrid Approach
April 3, 2023
Generally, the State-of-Health (SOH) monitoring and Remaining Useful Life (RUL) prediction and assessment of lithium-ion (Li-ion) batteries need to use sensors to obtain the degradation test data of the same type of batteries and establish the degradation model for reference. However, when the battery type is unknown, a usable reference model cannot be obtained, so its prediction and evaluation may be relatively inconvenient. In this paper, the State of-Health prediction for lithium-ion batteries based on a novel hybrid scheme is proposed. Firstly, historical charge/discharge time series and capacity series are extracted to analyze and construct Health Indicators, then using Complete Ensemble Empirical Mode Decomposition with Adaptive Noise (CEEMDAN) to decompose the Health Indicator series into the trend and non-trend terms. Among them, the relatively smooth trend item data series uses the Autoregressive Integrated Moving Average model (ARIMA) for prediction; when dealing with the data series of non-trend items which are obviously non-smooth and seemingly random, the residuals predicted by ARIMA and the non-trend items obtained by CEEMDAN decomposition are combined into new non-trend items; then the least square support vector machine (LSSVM) is introduced to build a nonlinear prediction model and make predictions. Finally, combining the prediction results of the trend item data series and the non-trend item data series as a reference for the assessment of the state of health and remaining useful life. The 13 experimental results of 3 batteries verify the effectiveness of the scheme.
Record ID
Keywords
ARIMA, Batteries, CEEMDAN, LSSVM, State-of-Health, Time Health Indicator
Subject
Suggested Citation
Yun Z, Qin W, Shi W, Ping P. State-of-Health Prediction for Lithium-Ion Batteries Based on a Novel Hybrid Approach. (2023). LAPSE:2023.26489
Author Affiliations
Yun Z: School of Instrument Science and Engineering, Southeast University, No. 2 Sipailou, Nanjing 210096, China
Qin W: School of Instrument Science and Engineering, Southeast University, No. 2 Sipailou, Nanjing 210096, China
Shi W: School of Instrument Science and Engineering, Southeast University, No. 2 Sipailou, Nanjing 210096, China
Ping P: School of Instrument Science and Engineering, Southeast University, No. 2 Sipailou, Nanjing 210096, China
Qin W: School of Instrument Science and Engineering, Southeast University, No. 2 Sipailou, Nanjing 210096, China
Shi W: School of Instrument Science and Engineering, Southeast University, No. 2 Sipailou, Nanjing 210096, China
Ping P: School of Instrument Science and Engineering, Southeast University, No. 2 Sipailou, Nanjing 210096, China
Journal Name
Energies
Volume
13
Issue
18
Article Number
E4858
Year
2020
Publication Date
2020-09-16
Published Version
ISSN
1996-1073
Version Comments
Original Submission
Other Meta
PII: en13184858, Publication Type: Journal Article
Record Map
Published Article
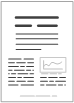
LAPSE:2023.26489
This Record
External Link
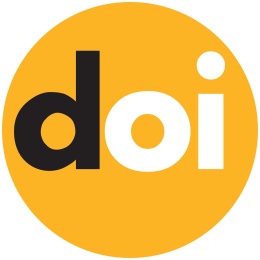
doi:10.3390/en13184858
Publisher Version
Download
Meta
Record Statistics
Record Views
105
Version History
[v1] (Original Submission)
Apr 3, 2023
Verified by curator on
Apr 3, 2023
This Version Number
v1
Citations
Most Recent
This Version
URL Here
https://psecommunity.org/LAPSE:2023.26489
Original Submitter
Auto Uploader for LAPSE
Links to Related Works