LAPSE:2023.25819
Published Article
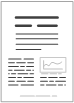
LAPSE:2023.25819
Multi-Horizon Forecasting of Global Horizontal Irradiance Using Online Gaussian Process Regression: A Kernel Study
March 29, 2023
In the present paper, global horizontal irradiance (GHI) is modelled and forecasted at time horizons ranging from 30 min to 48 h, thus covering intrahour, intraday and intraweek cases, using online Gaussian process regression (OGPR) and online sparse Gaussian process regression (OSGPR). The covariance function, also known as the kernel, is a key element that deeply influences forecasting accuracy. As a consequence, a comparative study of OGPR and OSGPR models based on simple kernels or combined kernels defined as sums or products of simple kernels has been carried out. The classic persistence model is included in the comparative study. Thanks to two datasets composed of GHI measurements (45 days), we have been able to show that OGPR models based on quasiperiodic kernels outperform the persistence model as well as OGPR models based on simple kernels, including the squared exponential kernel, which is widely used for GHI forecasting. Indeed, although all OGPR models give good results when the forecast horizon is short-term, when the horizon increases, the superiority of quasiperiodic kernels becomes apparent. A simple online sparse GPR (OSGPR) approach has also been assessed. This approach gives less precise results than standard GPR, but the training computation time is decreased to a great extent. Even though the lack of data hinders the training process, the results still show the superiority of GPR models based on quasiperiodic kernels for GHI forecasting.
Record ID
Keywords
global horizontal irradiance, Machine Learning, online Gaussian process regression, online sparse Gaussian process regression, solar resource, time series forecasting
Subject
Suggested Citation
Tolba H, Dkhili N, Nou J, Eynard J, Thil S, Grieu S. Multi-Horizon Forecasting of Global Horizontal Irradiance Using Online Gaussian Process Regression: A Kernel Study. (2023). LAPSE:2023.25819
Author Affiliations
Tolba H: PROMES-CNRS Laboratory (UPR 8521), Rambla de la Thermodynamique, Tecnosud, 66100 Perpignan, France
Dkhili N: PROMES-CNRS Laboratory (UPR 8521), Rambla de la Thermodynamique, Tecnosud, 66100 Perpignan, France; University of Perpignan Via Domitia, 52 avenue Paul Alduy, 66860 Perpignan, France
Nou J: PROMES-CNRS Laboratory (UPR 8521), Rambla de la Thermodynamique, Tecnosud, 66100 Perpignan, France
Eynard J: PROMES-CNRS Laboratory (UPR 8521), Rambla de la Thermodynamique, Tecnosud, 66100 Perpignan, France; University of Perpignan Via Domitia, 52 avenue Paul Alduy, 66860 Perpignan, France [ORCID]
Thil S: PROMES-CNRS Laboratory (UPR 8521), Rambla de la Thermodynamique, Tecnosud, 66100 Perpignan, France; University of Perpignan Via Domitia, 52 avenue Paul Alduy, 66860 Perpignan, France [ORCID]
Grieu S: PROMES-CNRS Laboratory (UPR 8521), Rambla de la Thermodynamique, Tecnosud, 66100 Perpignan, France; University of Perpignan Via Domitia, 52 avenue Paul Alduy, 66860 Perpignan, France
Dkhili N: PROMES-CNRS Laboratory (UPR 8521), Rambla de la Thermodynamique, Tecnosud, 66100 Perpignan, France; University of Perpignan Via Domitia, 52 avenue Paul Alduy, 66860 Perpignan, France
Nou J: PROMES-CNRS Laboratory (UPR 8521), Rambla de la Thermodynamique, Tecnosud, 66100 Perpignan, France
Eynard J: PROMES-CNRS Laboratory (UPR 8521), Rambla de la Thermodynamique, Tecnosud, 66100 Perpignan, France; University of Perpignan Via Domitia, 52 avenue Paul Alduy, 66860 Perpignan, France [ORCID]
Thil S: PROMES-CNRS Laboratory (UPR 8521), Rambla de la Thermodynamique, Tecnosud, 66100 Perpignan, France; University of Perpignan Via Domitia, 52 avenue Paul Alduy, 66860 Perpignan, France [ORCID]
Grieu S: PROMES-CNRS Laboratory (UPR 8521), Rambla de la Thermodynamique, Tecnosud, 66100 Perpignan, France; University of Perpignan Via Domitia, 52 avenue Paul Alduy, 66860 Perpignan, France
Journal Name
Energies
Volume
13
Issue
16
Article Number
E4184
Year
2020
Publication Date
2020-08-13
Published Version
ISSN
1996-1073
Version Comments
Original Submission
Other Meta
PII: en13164184, Publication Type: Journal Article
Record Map
Published Article
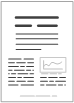
LAPSE:2023.25819
This Record
External Link
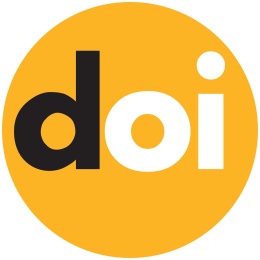
doi:10.3390/en13164184
Publisher Version
Download
Meta
Record Statistics
Record Views
110
Version History
[v1] (Original Submission)
Mar 29, 2023
Verified by curator on
Mar 29, 2023
This Version Number
v1
Citations
Most Recent
This Version
URL Here
https://psecommunity.org/LAPSE:2023.25819
Original Submitter
Auto Uploader for LAPSE
Links to Related Works