LAPSE:2023.25793
Published Article
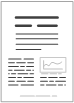
LAPSE:2023.25793
Multi-Label Learning for Appliance Recognition in NILM Using Fryze-Current Decomposition and Convolutional Neural Network
March 29, 2023
The advance in energy-sensing and smart-meter technologies have motivated the use of a Non-Intrusive Load Monitoring (NILM), a data-driven technique that recognizes active end-use appliances by analyzing the data streams coming from these devices. NILM offers an electricity consumption pattern of individual loads at consumer premises, which is crucial in the design of energy efficiency and energy demand management strategies in buildings. Appliance classification, also known as load identification is an essential sub-task for identifying the type and status of an unknown load from appliance features extracted from the aggregate power signal. Most of the existing work for appliance recognition in NILM uses a single-label learning strategy which, assumes only one appliance is active at a time. This assumption ignores the fact that multiple devices can be active simultaneously and requires a perfect event detector to recognize the appliance. In this paper proposes the Convolutional Neural Network (CNN)-based multi-label learning approach, which links multiple loads to an observed aggregate current signal. Our approach applies the Fryze power theory to decompose the current features into active and non-active components and use the Euclidean distance similarity function to transform the decomposed current into an image-like representation which, is used as input to the CNN. Experimental results suggest that the proposed approach is sufficient for recognizing multiple appliances from aggregated measurements.
Record ID
Keywords
activation current, appliance recognition, Convolutional Neural Network, distance similarity matrix, fryze power theory, multi-label learning, Non-intrusive Load Monitoring, V-I trajectory
Suggested Citation
Faustine A, Pereira L. Multi-Label Learning for Appliance Recognition in NILM Using Fryze-Current Decomposition and Convolutional Neural Network. (2023). LAPSE:2023.25793
Author Affiliations
Journal Name
Energies
Volume
13
Issue
16
Article Number
E4154
Year
2020
Publication Date
2020-08-11
Published Version
ISSN
1996-1073
Version Comments
Original Submission
Other Meta
PII: en13164154, Publication Type: Journal Article
Record Map
Published Article
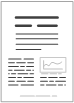
LAPSE:2023.25793
This Record
External Link
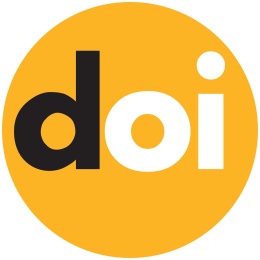
doi:10.3390/en13164154
Publisher Version
Download
Meta
Record Statistics
Record Views
57
Version History
[v1] (Original Submission)
Mar 29, 2023
Verified by curator on
Mar 29, 2023
This Version Number
v1
Citations
Most Recent
This Version
URL Here
https://psecommunity.org/LAPSE:2023.25793
Original Submitter
Auto Uploader for LAPSE
Links to Related Works