LAPSE:2023.25677
Published Article
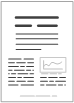
LAPSE:2023.25677
A Methodology for Energy Load Profile Forecasting Based on Intelligent Clustering and Smoothing Techniques
March 29, 2023
The electrical sector needs to study how energy demand changes to plan the maintenance and purchase of energy assets properly. Prediction studies for energy demand require a high level of reliability since a deviation in the forecasting demand could affect operation costs. This paper proposed a short-term forecasting energy demand methodology based on hierarchical clustering using Dynamic Time Warp as a similarity measure integrated with Artificial Neural Networks. Clustering was used to build the typical curve for each type of day, while Artificial Neural Networks handled the weather sensibility to correct a preliminary forecasting curve obtained in the clustering stage. A statistical analysis was carried out to identify those significant factors in the prediction model of energy demand. The performance of this proposed model was measured through the Mean Absolute Percentage Error (MAPE). The experimental results show that the three-stage methodology was able to improve the MAPE, reaching values as good as 2%.
Record ID
Keywords
artificial neural networks, clustering, demand forecasting, time series analysis
Suggested Citation
Jiménez Mares J, Navarro L, Quintero M. CG, Pardo M. A Methodology for Energy Load Profile Forecasting Based on Intelligent Clustering and Smoothing Techniques. (2023). LAPSE:2023.25677
Author Affiliations
Jiménez Mares J: Department of Electrical and Electronics Engineering; Universidad del Norte, Barranquilla 081007, Colombia [ORCID]
Navarro L: Department of Electrical and Electronics Engineering; Universidad del Norte, Barranquilla 081007, Colombia [ORCID]
Quintero M. CG: Department of Electrical and Electronics Engineering; Universidad del Norte, Barranquilla 081007, Colombia [ORCID]
Pardo M: Department of Electrical and Electronics Engineering; Universidad del Norte, Barranquilla 081007, Colombia [ORCID]
Navarro L: Department of Electrical and Electronics Engineering; Universidad del Norte, Barranquilla 081007, Colombia [ORCID]
Quintero M. CG: Department of Electrical and Electronics Engineering; Universidad del Norte, Barranquilla 081007, Colombia [ORCID]
Pardo M: Department of Electrical and Electronics Engineering; Universidad del Norte, Barranquilla 081007, Colombia [ORCID]
Journal Name
Energies
Volume
13
Issue
16
Article Number
E4040
Year
2020
Publication Date
2020-08-05
Published Version
ISSN
1996-1073
Version Comments
Original Submission
Other Meta
PII: en13164040, Publication Type: Journal Article
Record Map
Published Article
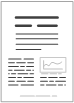
LAPSE:2023.25677
This Record
External Link
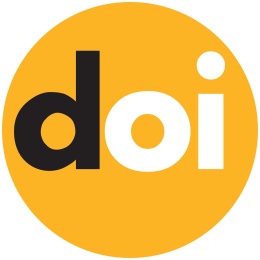
doi:10.3390/en13164040
Publisher Version
Download
Meta
Record Statistics
Record Views
57
Version History
[v1] (Original Submission)
Mar 29, 2023
Verified by curator on
Mar 29, 2023
This Version Number
v1
Citations
Most Recent
This Version
URL Here
https://psecommunity.org/LAPSE:2023.25677
Original Submitter
Auto Uploader for LAPSE
Links to Related Works