LAPSE:2023.23183
Published Article
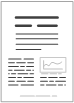
LAPSE:2023.23183
Machine Learning Modeling of Horizontal Photovoltaics Using Weather and Location Data
March 27, 2023
Solar energy is a key renewable energy source; however, its intermittent nature and potential for use in distributed systems make power prediction an important aspect of grid integration. This research analyzed a variety of machine learning techniques to predict power output for horizontal solar panels using 14 months of data collected from 12 northern-hemisphere locations. We performed our data collection and analysis in the absence of irradiation data—an approach not commonly found in prior literature. Using latitude, month, hour, ambient temperature, pressure, humidity, wind speed, and cloud ceiling as independent variables, a distributed random forest regression algorithm modeled the combined dataset with an R2 value of 0.94. As a comparative measure, other machine learning algorithms resulted in R2 values of 0.50−0.94. Additionally, the data from each location was modeled separately with R2 values ranging from 0.91 to 0.97, indicating a range of consistency across all sites. Using an input variable permutation approach with the random forest algorithm, we found that the three most important variables for power prediction were ambient temperature, humidity, and cloud ceiling. The analysis showed that machine learning potentially allowed for accurate power prediction while avoiding the challenges associated with modeled irradiation data.
Record ID
Keywords
Machine Learning, photovoltaics, power prediction, random forest, Solar Panels
Subject
Suggested Citation
Pasion C, Wagner T, Koschnick C, Schuldt S, Williams J, Hallinan K. Machine Learning Modeling of Horizontal Photovoltaics Using Weather and Location Data. (2023). LAPSE:2023.23183
Author Affiliations
Pasion C: Graduate School of Engineering and Management, Air Force Institute of Technology, Wright-Patterson AFB, OH 45433, USA
Wagner T: Graduate School of Engineering and Management, Air Force Institute of Technology, Wright-Patterson AFB, OH 45433, USA [ORCID]
Koschnick C: Graduate School of Engineering and Management, Air Force Institute of Technology, Wright-Patterson AFB, OH 45433, USA
Schuldt S: Graduate School of Engineering and Management, Air Force Institute of Technology, Wright-Patterson AFB, OH 45433, USA [ORCID]
Williams J: Graduate School of Engineering and Management, Air Force Institute of Technology, Wright-Patterson AFB, OH 45433, USA
Hallinan K: Department of Mechanical and Aerospace Engineering, University of Dayton, Dayton, OH 45469, USA [ORCID]
Wagner T: Graduate School of Engineering and Management, Air Force Institute of Technology, Wright-Patterson AFB, OH 45433, USA [ORCID]
Koschnick C: Graduate School of Engineering and Management, Air Force Institute of Technology, Wright-Patterson AFB, OH 45433, USA
Schuldt S: Graduate School of Engineering and Management, Air Force Institute of Technology, Wright-Patterson AFB, OH 45433, USA [ORCID]
Williams J: Graduate School of Engineering and Management, Air Force Institute of Technology, Wright-Patterson AFB, OH 45433, USA
Hallinan K: Department of Mechanical and Aerospace Engineering, University of Dayton, Dayton, OH 45469, USA [ORCID]
Journal Name
Energies
Volume
13
Issue
10
Article Number
E2570
Year
2020
Publication Date
2020-05-19
Published Version
ISSN
1996-1073
Version Comments
Original Submission
Other Meta
PII: en13102570, Publication Type: Journal Article
Record Map
Published Article
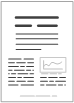
LAPSE:2023.23183
This Record
External Link
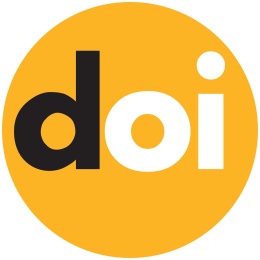
doi:10.3390/en13102570
Publisher Version
Download
Meta
Record Statistics
Record Views
123
Version History
[v1] (Original Submission)
Mar 27, 2023
Verified by curator on
Mar 27, 2023
This Version Number
v1
Citations
Most Recent
This Version
URL Here
https://psecommunity.org/LAPSE:2023.23183
Original Submitter
Auto Uploader for LAPSE
Links to Related Works