LAPSE:2023.22451
Published Article
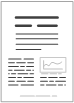
LAPSE:2023.22451
Research on Parameter Self-Learning Unscented Kalman Filtering Algorithm and Its Application in Battery Charge of State Estimation
March 24, 2023
A novel state estimation algorithm based on the parameters of a self-learning unscented Kalman filter (UKF) with a model parameter identification method based on a collaborative optimization mechanism is proposed in this paper. This algorithm can realize the dynamic self-learning and self-adjustment of the parameters in the UKF algorithm and the automatic optimization setting Sigma points without human participation. In addition, the multi-algorithm collaborative optimization mechanism unifies a variety of algorithms, so that the identification method has the advantages of member algorithms while avoiding the disadvantages of them. We apply the combination algorithm proposed in this paper for state of charge (SoC) estimation of power batteries and compare it with other model parameter identification algorithms and SoC estimation methods. The results showed that the proposed algorithm outperformed the other model parameter identification algorithms in terms of estimation accuracy and robustness.
Record ID
Keywords
battery management system, parameter identification, state of charge, unscented Kalman filter
Subject
Suggested Citation
Liu F, Ma J, Su W, Chen H, He M. Research on Parameter Self-Learning Unscented Kalman Filtering Algorithm and Its Application in Battery Charge of State Estimation. (2023). LAPSE:2023.22451
Author Affiliations
Liu F: School of Computer Science & Technology, Tiangong University, Tianjin 300387, China [ORCID]
Ma J: School of Computer Science & Technology, Tiangong University, Tianjin 300387, China
Su W: School of Computer Science & Technology, Tiangong University, Tianjin 300387, China; State Key Laboratory of Process Automation in Mining & Metallurgy, Beijing 100160, China; Beijing Key Laboratory of Process Automation in Mining & Metallurgy, Beijing 100
Chen H: School of Computer Science & Technology, Tiangong University, Tianjin 300387, China
He M: School of Computer Science & Technology, Tiangong University, Tianjin 300387, China
Ma J: School of Computer Science & Technology, Tiangong University, Tianjin 300387, China
Su W: School of Computer Science & Technology, Tiangong University, Tianjin 300387, China; State Key Laboratory of Process Automation in Mining & Metallurgy, Beijing 100160, China; Beijing Key Laboratory of Process Automation in Mining & Metallurgy, Beijing 100
Chen H: School of Computer Science & Technology, Tiangong University, Tianjin 300387, China
He M: School of Computer Science & Technology, Tiangong University, Tianjin 300387, China
Journal Name
Energies
Volume
13
Issue
7
Article Number
E1679
Year
2020
Publication Date
2020-04-03
Published Version
ISSN
1996-1073
Version Comments
Original Submission
Other Meta
PII: en13071679, Publication Type: Journal Article
Record Map
Published Article
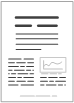
LAPSE:2023.22451
This Record
External Link
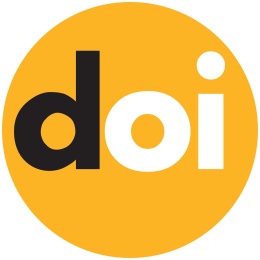
doi:10.3390/en13071679
Publisher Version
Download
Meta
Record Statistics
Record Views
74
Version History
[v1] (Original Submission)
Mar 24, 2023
Verified by curator on
Mar 24, 2023
This Version Number
v1
Citations
Most Recent
This Version
URL Here
https://psecommunity.org/LAPSE:2023.22451
Original Submitter
Auto Uploader for LAPSE
Links to Related Works