LAPSE:2023.21739
Published Article
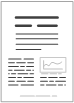
LAPSE:2023.21739
Surrogate Model with a Deep Neural Network to Evaluate Gas−Liquid Flow in a Horizontal Pipe
March 23, 2023
This study developed a data-driven surrogate model based on a deep neural network (DNN) to evaluate gas−liquid multiphase flow occurring in horizontal pipes. It estimated the liquid holdup and pressure gradient under a slip condition and different flow patterns, i.e., slug, annular, stratified flow, etc. The inputs of the surrogate modelling were related to the fluid properties and the dynamic data, e.g., superficial velocities at the inlet, while the outputs were the liquid holdup and pressure gradient observed at the outlet. The case study determined the optimal number of hidden neurons by considering the processing time and the validation error. A total of 350 experimental data were used: 279 for supervised training, 31 for validating the training performance, and 40 unknown data, not used in training and validation, were examined to forecast the liquid holdup and pressure gradient. The liquid holdups were estimated within less than 8.08% of the mean absolute percentage error, while the error of the pressure gradient was 23.76%. The R2 values confirmed the reliability of the developed model, showing 0.89 for liquid holdups and 0.98 for pressure gradients. The DNN-based surrogate model can be applicable to estimate liquid holdup and pressure gradients in a more realistic manner with a small amount of computating resources.
Record ID
Keywords
deep neural network, horizontal pipe, liquid holdup, multiphase flow, pressure gradient, Surrogate Model
Suggested Citation
Seong Y, Park C, Choi J, Jang I. Surrogate Model with a Deep Neural Network to Evaluate Gas−Liquid Flow in a Horizontal Pipe. (2023). LAPSE:2023.21739
Author Affiliations
Seong Y: Department of Energy and Resources Engineering, Kangwon National University, Chuncheon, Kangwon 24341, Korea
Park C: Department of Energy and Resources Engineering, Kangwon National University, Chuncheon, Kangwon 24341, Korea [ORCID]
Choi J: Naval & Energy System R&D Institute, Daewoo Shipbuilding & Marine Engineering Co., Ltd., Siheung, Gyeonggi 15011, Korea
Jang I: Department of Energy and Resources Engineering, Chosun University, Gwangju 61425, Korea
Park C: Department of Energy and Resources Engineering, Kangwon National University, Chuncheon, Kangwon 24341, Korea [ORCID]
Choi J: Naval & Energy System R&D Institute, Daewoo Shipbuilding & Marine Engineering Co., Ltd., Siheung, Gyeonggi 15011, Korea
Jang I: Department of Energy and Resources Engineering, Chosun University, Gwangju 61425, Korea
Journal Name
Energies
Volume
13
Issue
4
Article Number
E968
Year
2020
Publication Date
2020-02-21
Published Version
ISSN
1996-1073
Version Comments
Original Submission
Other Meta
PII: en13040968, Publication Type: Journal Article
Record Map
Published Article
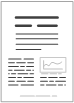
LAPSE:2023.21739
This Record
External Link
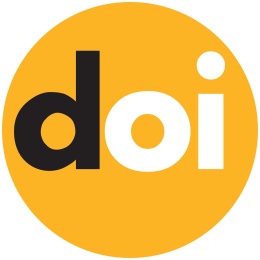
doi:10.3390/en13040968
Publisher Version
Download
Meta
Record Statistics
Record Views
111
Version History
[v1] (Original Submission)
Mar 23, 2023
Verified by curator on
Mar 23, 2023
This Version Number
v1
Citations
Most Recent
This Version
URL Here
https://psecommunity.org/LAPSE:2023.21739
Original Submitter
Auto Uploader for LAPSE
Links to Related Works