LAPSE:2023.21644
Published Article
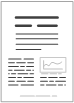
LAPSE:2023.21644
A Multivariate Statistics-Based Approach for Detecting Diesel Engine Faults with Weak Signatures
March 22, 2023
The problem of timely detecting the engine faults that make engine operating parameters exceed their control limits has been well-solved. However, in practice, a fault of a diesel engine can be present with weak signatures, with the parameters fluctuating within their control limits when the fault occurs. The weak signatures of engine faults bring considerable difficulties to the effective condition monitoring of diesel engines. In this paper, a multivariate statistics-based fault detection approach is proposed to monitor engine faults with weak signatures by taking the correlation of various parameters into consideration. This approach firstly uses principal component analysis (PCA) to project the engine observations into a principal component subspace (PCS) and a residual subspace (RS). Two statistics, i.e., Hotelling’s T 2 and Q statistics, are then introduced to detect deviations in the PCS and the RS, respectively. The Hotelling’s T 2 and Q statistics are constructed by taking the correlation of various parameters into consideration, so that faults with weak signatures can be effectively detected via these two statistics. In order to reasonably determine the control limits of the statistics, adaptive kernel density estimation (KDE) is utilized to estimate the probability density functions (PDFs) of Hotelling’s T 2 and Q statistics. The control limits are accordingly derived from the PDFs by giving a desired confidence level. The proposed approach is demonstrated by using a marine diesel engine. Experimental results show that the proposed approach can effectively detect engine faults with weak signatures.
Record ID
Keywords
condition monitoring, diesel engine, Fault Detection, Multivariate Statistics, principal component analysis
Suggested Citation
Wang J, Zhang C, Ma X, Wang Z, Xu Y, Cattley R. A Multivariate Statistics-Based Approach for Detecting Diesel Engine Faults with Weak Signatures. (2023). LAPSE:2023.21644
Author Affiliations
Wang J: College of Power and Energy Engineering, Harbin Engineering University, Harbin 150001, China
Zhang C: College of Power and Energy Engineering, Harbin Engineering University, Harbin 150001, China
Ma X: College of Power and Energy Engineering, Harbin Engineering University, Harbin 150001, China
Wang Z: College of Power and Energy Engineering, Harbin Engineering University, Harbin 150001, China
Xu Y: Centre for Efficiency and Performance Engineering, University of Huddersfield, Huddersfield HD1 3DH, UK [ORCID]
Cattley R: Centre for Efficiency and Performance Engineering, University of Huddersfield, Huddersfield HD1 3DH, UK
Zhang C: College of Power and Energy Engineering, Harbin Engineering University, Harbin 150001, China
Ma X: College of Power and Energy Engineering, Harbin Engineering University, Harbin 150001, China
Wang Z: College of Power and Energy Engineering, Harbin Engineering University, Harbin 150001, China
Xu Y: Centre for Efficiency and Performance Engineering, University of Huddersfield, Huddersfield HD1 3DH, UK [ORCID]
Cattley R: Centre for Efficiency and Performance Engineering, University of Huddersfield, Huddersfield HD1 3DH, UK
Journal Name
Energies
Volume
13
Issue
4
Article Number
E873
Year
2020
Publication Date
2020-02-17
Published Version
ISSN
1996-1073
Version Comments
Original Submission
Other Meta
PII: en13040873, Publication Type: Journal Article
Record Map
Published Article
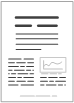
LAPSE:2023.21644
This Record
External Link
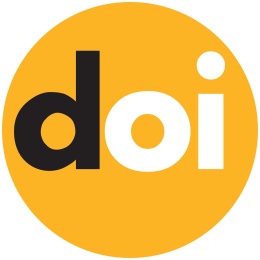
doi:10.3390/en13040873
Publisher Version
Download
Meta
Record Statistics
Record Views
167
Version History
[v1] (Original Submission)
Mar 22, 2023
Verified by curator on
Mar 22, 2023
This Version Number
v1
Citations
Most Recent
This Version
URL Here
https://psecommunity.org/LAPSE:2023.21644
Original Submitter
Auto Uploader for LAPSE
Links to Related Works