LAPSE:2023.21568
Published Article
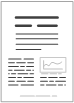
LAPSE:2023.21568
Multi-State Household Appliance Identification Based on Convolutional Neural Networks and Clustering
March 22, 2023
Non-intrusive load monitoring, a convenient way to discern the energy consumption of a house, has been studied extensively. However, most research works have been carried out based on a hypothetical condition that each electric appliance has only one running state. This leads to low identification accuracy for multi-state electric appliances. To deal with this problem, a method for identifying the type and state of electric appliances based on a power time series is proposed in this paper. First, to identify the type of appliance, a convolutional neural network model was constructed that incorporated residual modules. Then, a k-means clustering algorithm was applied to calculate the number of states of the appliance. Finally, in order to identify the states of the appliances, different k-means clustering models were established for different multi-state electric appliances. Experimental results show effectiveness of the proposed method in identifying both the type and the running state of electric appliances.
Record ID
Keywords
non-intrusive load monitoring, the identification of appliances states, the identification of appliances types
Subject
Suggested Citation
Zhang Y, Yin B, Cong Y, Du Z. Multi-State Household Appliance Identification Based on Convolutional Neural Networks and Clustering. (2023). LAPSE:2023.21568
Author Affiliations
Zhang Y: College of Information Science and Engineering, Ocean University of China, Qingdao 266000, China [ORCID]
Yin B: College of Information Science and Engineering, Ocean University of China, Qingdao 266000, China; Pilot National Laboratory for Marine Science and Technology, Qingdao 266000, China
Cong Y: College of Information Science and Engineering, Ocean University of China, Qingdao 266000, China
Du Z: College of Information Science and Engineering, Ocean University of China, Qingdao 266000, China
Yin B: College of Information Science and Engineering, Ocean University of China, Qingdao 266000, China; Pilot National Laboratory for Marine Science and Technology, Qingdao 266000, China
Cong Y: College of Information Science and Engineering, Ocean University of China, Qingdao 266000, China
Du Z: College of Information Science and Engineering, Ocean University of China, Qingdao 266000, China
Journal Name
Energies
Volume
13
Issue
4
Article Number
E792
Year
2020
Publication Date
2020-02-11
Published Version
ISSN
1996-1073
Version Comments
Original Submission
Other Meta
PII: en13040792, Publication Type: Journal Article
Record Map
Published Article
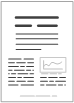
LAPSE:2023.21568
This Record
External Link
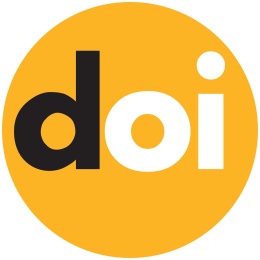
doi:10.3390/en13040792
Publisher Version
Download
Meta
Record Statistics
Record Views
69
Version History
[v1] (Original Submission)
Mar 22, 2023
Verified by curator on
Mar 22, 2023
This Version Number
v1
Citations
Most Recent
This Version
URL Here
https://psecommunity.org/LAPSE:2023.21568
Original Submitter
Auto Uploader for LAPSE
Links to Related Works