LAPSE:2023.21154
Published Article
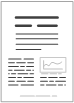
LAPSE:2023.21154
Sensor Data Compression Using Bounded Error Piecewise Linear Approximation with Resolution Reduction
March 21, 2023
Smart production as one of the key issues for the world to advance toward Industry 4.0 has been a research focus in recent years. In a smart factory, hundreds or even thousands of sensors and smart devices are often deployed to enhance product quality. Generally, sensor data provides abundant information for artificial intelligence (AI) engines to make decisions for these smart devices to collect more data or activate some required activities. However, this also consumes a lot of energy to transmit the sensor data via networks and store them in data centers. Data compression is a common approach to reduce the sensor data size so as to lower transmission energies. Literature indicates that many Bounded-Error Piecewise Linear Approximation (BEPLA) methods have been proposed to achieve this. Given an error bound, they make efforts on how to approximate to the original sensor data with fewer line segments. In this paper, we furthermore consider resolution reduction, which sets a new restriction on the position of line segment endpoints. Swing-RR (Resolution Reduction) is then proposed. It has O(1) complexity in both space and time per data record. In other words, Swing-RR is suitable for compressing sensor data, particularly when the volume of the data is huge. Our experimental results on real world datasets show that the size of compressed data is significantly reduced. The energy consumed follows. When using minimal resolution, Swing-RR has achieved the best compression ratios for all tested datasets. Consequently, fewer bits are transmitted through networks and less disk space is required to store the data in data centers, thus consuming less data transmission and storage power.
Record ID
Keywords
Big Data, bounded-error approximation, data compression, Internet of Things, piecewise linear, resolution reduction
Subject
Suggested Citation
Lin JW, Liao SW, Leu FY. Sensor Data Compression Using Bounded Error Piecewise Linear Approximation with Resolution Reduction. (2023). LAPSE:2023.21154
Author Affiliations
Lin JW: Department of Information Management, Tunghai University, Taichung 40704, Taiwan
Liao SW: Department of Computer Science and Information Engineering, National Taiwan University, Taipei 10617, Taiwan
Leu FY: Department of Computer Science, Tunghai University, Taichung 40704, Taiwan
Liao SW: Department of Computer Science and Information Engineering, National Taiwan University, Taipei 10617, Taiwan
Leu FY: Department of Computer Science, Tunghai University, Taichung 40704, Taiwan
Journal Name
Energies
Volume
12
Issue
13
Article Number
E2523
Year
2019
Publication Date
2019-06-30
Published Version
ISSN
1996-1073
Version Comments
Original Submission
Other Meta
PII: en12132523, Publication Type: Journal Article
Record Map
Published Article
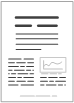
LAPSE:2023.21154
This Record
External Link
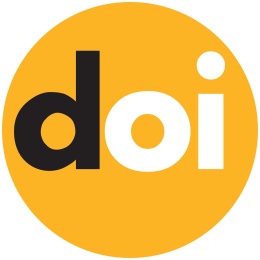
doi:10.3390/en12132523
Publisher Version
Download
Meta
Record Statistics
Record Views
122
Version History
[v1] (Original Submission)
Mar 21, 2023
Verified by curator on
Mar 21, 2023
This Version Number
v1
Citations
Most Recent
This Version
URL Here
https://psecommunity.org/LAPSE:2023.21154
Original Submitter
Auto Uploader for LAPSE
Links to Related Works