LAPSE:2023.20640
Published Article
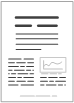
LAPSE:2023.20640
Comprehensive Analysis of Solid Oxide Fuel Cell Performance Degradation Mechanism, Prediction, and Optimization Studies
March 20, 2023
Solid oxide fuel cell (SOFC) performance degradation analysis and optimization studies are important prerequisites for its commercialization. Reviewing and summarizing SOFC performance degradation studies can help researchers identify research gaps and increase investment in weak areas. In this study, to help researchers purposely improve system performance, degradation mechanism analysis, degradation performance prediction, and degradation performance optimization studies are sorted out. In the review, it is found that the degradation mechanism analysis studies can help to improve the system structure. Degradation mechanism analysis studies can be performed at the stack level and system level, respectively. Degradation performance prediction can help to take measures to mitigate degradation in advance. The main tools of prediction study can be divided into model-based, data-based, electrochemical impedance spectroscopy-based, and image-based approaches. Degradation performance optimization can improve the system performance based on degradation mechanism analysis and performance prediction results. The optimization study focuses on two aspects of constitutive improvement and health controller design. However, the existing research is not yet complete. In-depth studies on performance degradation are still needed to achieve further SOFC commercialization. This paper summarizes mainstream research methods, as well as deficiencies that can provide partial theoretical guidance for SOFC performance enhancement.
Record ID
Keywords
degradation mechanism analysis, degradation performance optimization, degradation performance prediction, Solid Oxide Fuel Cells
Subject
Suggested Citation
Peng J, Zhao D, Xu Y, Wu X, Li X. Comprehensive Analysis of Solid Oxide Fuel Cell Performance Degradation Mechanism, Prediction, and Optimization Studies. (2023). LAPSE:2023.20640
Author Affiliations
Peng J: School of Artificial Intelligence and Automation, Key Laboratory of Imaging Processing and Intelligent Control of Education Ministry, Huazhong University of Science and Technology, Wuhan 430074, China [ORCID]
Zhao D: School of Artificial Intelligence and Automation, Key Laboratory of Imaging Processing and Intelligent Control of Education Ministry, Huazhong University of Science and Technology, Wuhan 430074, China
Xu Y: School of Information Science and Engineering, Wuhan University of Science and Technology, Wuhan 430081, China
Wu X: School of Information Engineering, Nanchang University, Nanchang 330031, China
Li X: School of Artificial Intelligence and Automation, Key Laboratory of Imaging Processing and Intelligent Control of Education Ministry, Huazhong University of Science and Technology, Wuhan 430074, China; Shenzhen Huazhong University of Science and Technolog
Zhao D: School of Artificial Intelligence and Automation, Key Laboratory of Imaging Processing and Intelligent Control of Education Ministry, Huazhong University of Science and Technology, Wuhan 430074, China
Xu Y: School of Information Science and Engineering, Wuhan University of Science and Technology, Wuhan 430081, China
Wu X: School of Information Engineering, Nanchang University, Nanchang 330031, China
Li X: School of Artificial Intelligence and Automation, Key Laboratory of Imaging Processing and Intelligent Control of Education Ministry, Huazhong University of Science and Technology, Wuhan 430074, China; Shenzhen Huazhong University of Science and Technolog
Journal Name
Energies
Volume
16
Issue
2
First Page
788
Year
2023
Publication Date
2023-01-10
Published Version
ISSN
1996-1073
Version Comments
Original Submission
Other Meta
PII: en16020788, Publication Type: Review
Record Map
Published Article
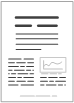
LAPSE:2023.20640
This Record
External Link
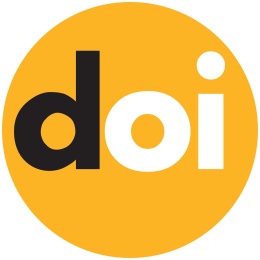
doi:10.3390/en16020788
Publisher Version
Download
Meta
Record Statistics
Record Views
110
Version History
[v1] (Original Submission)
Mar 20, 2023
Verified by curator on
Mar 20, 2023
This Version Number
v1
Citations
Most Recent
This Version
URL Here
https://psecommunity.org/LAPSE:2023.20640
Original Submitter
Auto Uploader for LAPSE
Links to Related Works