LAPSE:2023.17518
Published Article
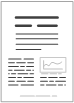
LAPSE:2023.17518
A Multi-Agent Reinforcement Learning Approach to Price and Comfort Optimization in HVAC-Systems
March 6, 2023
This paper addresses the challenge of minimizing training time for the control of Heating, Ventilation, and Air-conditioning (HVAC) systems with online Reinforcement Learning (RL). This is done by developing a novel approach to Multi-Agent Reinforcement Learning (MARL) to HVAC systems. In this paper, the environment formed by the HVAC system is formulated as a Markov Game (MG) in a general sum setting. The MARL algorithm is designed in a decentralized structure, where only relevant states are shared between agents, and actions are shared in a sequence, which are sensible from a system’s point of view. The simulation environment is a domestic house located in Denmark and designed to resemble an average house. The heat source in the house is an air-to-water heat pump, and the HVAC system is an Underfloor Heating system (UFH). The house is subjected to weather changes from a data set collected in Copenhagen in 2006, spanning the entire year except for June, July, and August, where heat is not required. It is shown that: (1) When comparing Single Agent Reinforcement Learning (SARL) and MARL, training time can be reduced by 70% for a four temperature-zone UFH system, (2) the agent can learn and generalize over seasons, (3) the cost of heating can be reduced by 19% or the equivalent to 750 kWh of electric energy per year for an average Danish domestic house compared to a traditional control method, and (4) oscillations in the room temperature can be reduced by 40% when comparing the RL control methods with a traditional control method.
Record ID
Keywords
Artificial Intelligence, deep reinforcement learning, energy in buildings, HVAC-systems, predictive analytics, underfloor heating
Subject
Suggested Citation
Blad C, Bøgh S, Kallesøe C. A Multi-Agent Reinforcement Learning Approach to Price and Comfort Optimization in HVAC-Systems. (2023). LAPSE:2023.17518
Author Affiliations
Blad C: Robotics & Automation Group, Department of Materials and Production, Aalborg University, 9220 Aalborg, Denmark; Technology and Innovation, Control Department, Grundfos, 8850 Bjerringbro, Denmark [ORCID]
Bøgh S: Robotics & Automation Group, Department of Materials and Production, Aalborg University, 9220 Aalborg, Denmark
Kallesøe C: Technology and Innovation, Control Department, Grundfos, 8850 Bjerringbro, Denmark; Department of Electronic Systems, Aalborg University, 9220 Aalborg, Denmark [ORCID]
Bøgh S: Robotics & Automation Group, Department of Materials and Production, Aalborg University, 9220 Aalborg, Denmark
Kallesøe C: Technology and Innovation, Control Department, Grundfos, 8850 Bjerringbro, Denmark; Department of Electronic Systems, Aalborg University, 9220 Aalborg, Denmark [ORCID]
Journal Name
Energies
Volume
14
Issue
22
First Page
7491
Year
2021
Publication Date
2021-11-09
Published Version
ISSN
1996-1073
Version Comments
Original Submission
Other Meta
PII: en14227491, Publication Type: Journal Article
Record Map
Published Article
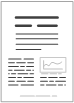
LAPSE:2023.17518
This Record
External Link
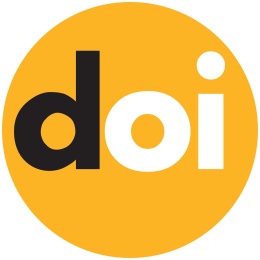
doi:10.3390/en14227491
Publisher Version
Download
Meta
Record Statistics
Record Views
102
Version History
[v1] (Original Submission)
Mar 6, 2023
Verified by curator on
Mar 6, 2023
This Version Number
v1
Citations
Most Recent
This Version
URL Here
https://psecommunity.org/LAPSE:2023.17518
Original Submitter
Auto Uploader for LAPSE
Links to Related Works