LAPSE:2023.17462
Published Article
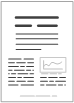
LAPSE:2023.17462
A QoS-Aware Machine Learning-Based Framework for AMI Applications in Smart Grids
March 6, 2023
The Internet of things (IoT) enables a diverse set of applications such as distribution automation, smart cities, wireless sensor networks, and advanced metering infrastructure (AMI). In smart grids (SGs), quality of service (QoS) and AMI traffic management need to be considered in the design of efficient AMI architectures. In this article, we propose a QoS-aware machine-learning-based framework for AMI applications in smart grids. Our proposed framework comprises a three-tier hierarchical architecture for AMI applications, a machine-learning-based hierarchical clustering approach, and a priority-based scheduling technique to ensure QoS in AMI applications in smart grids. We introduce a three-tier hierarchical architecture for AMI applications in smart grids to take advantage of IoT communication technologies and the cloud infrastructure. In this architecture, smart meters are deployed over a georeferenced area where the control center has remote access over the Internet to these network devices. More specifically, these devices can be digitally controlled and monitored using simple web interfaces such as REST APIs. We modify the existing K-means algorithm to construct a hierarchical clustering topology that employs Wi-SUN technology for bi-directional communication between smart meters and data concentrators. Further, we develop a queuing model in which different priorities are assigned to each item of the critical and normal AMI traffic based on its latency and packet size. The critical AMI traffic is scheduled first using priority-based scheduling while the normal traffic is scheduled with a first-in−first-out scheduling scheme to ensure the QoS requirements of both traffic classes in the smart grid network. The numerical results demonstrate that the target coverage and connectivity requirements of all smart meters are fulfilled with the least number of data concentrators in the design. Additionally, the numerical results show that the architectural cost is reduced, and the bottleneck problem of the data concentrator is eliminated. Furthermore, the performance of the proposed framework is evaluated and validated on the CloudSim simulator. The simulation results of our proposed framework show efficient performance in terms of CPU utilization compared to a traditional framework that uses single-hop communication from smart meters to data concentrators with a first-in−first-out scheduling scheme.
Record ID
Keywords
advanced metering infrastructure (AMI), aggregator head (AH), CloudSim, cluster head (CH), coverage, first-in–first-out (FIFO), Internet of things (IoT), K-means, latency, quality of service (QoS), smart grid (SG)
Subject
Suggested Citation
Khan A, Umar AI, Munir A, Shirazi SH, Khan MA, Adnan M. A QoS-Aware Machine Learning-Based Framework for AMI Applications in Smart Grids. (2023). LAPSE:2023.17462
Author Affiliations
Khan A: Department of Information Technology, Hazara University Mansehra, Mansehra 21120, Pakistan
Umar AI: Department of Information Technology, Hazara University Mansehra, Mansehra 21120, Pakistan
Munir A: Intelligent Systems, Computer Architecture, Analytics, and Security (ISCAAS) Laboratory, Department of Computer Science, Kansas State University, Manhattan, KA 66506, USA [ORCID]
Shirazi SH: Department of Information Technology, Hazara University Mansehra, Mansehra 21120, Pakistan [ORCID]
Khan MA: Department of Computer Science, Quid-i-Azam University, Islamabad 44000, Pakistan [ORCID]
Adnan M: Department of Information Technology, Hazara University Mansehra, Mansehra 21120, Pakistan
Umar AI: Department of Information Technology, Hazara University Mansehra, Mansehra 21120, Pakistan
Munir A: Intelligent Systems, Computer Architecture, Analytics, and Security (ISCAAS) Laboratory, Department of Computer Science, Kansas State University, Manhattan, KA 66506, USA [ORCID]
Shirazi SH: Department of Information Technology, Hazara University Mansehra, Mansehra 21120, Pakistan [ORCID]
Khan MA: Department of Computer Science, Quid-i-Azam University, Islamabad 44000, Pakistan [ORCID]
Adnan M: Department of Information Technology, Hazara University Mansehra, Mansehra 21120, Pakistan
Journal Name
Energies
Volume
14
Issue
23
First Page
8171
Year
2021
Publication Date
2021-12-06
Published Version
ISSN
1996-1073
Version Comments
Original Submission
Other Meta
PII: en14238171, Publication Type: Journal Article
Record Map
Published Article
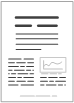
LAPSE:2023.17462
This Record
External Link
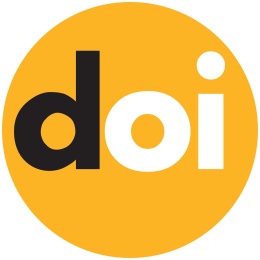
doi:10.3390/en14238171
Publisher Version
Download
Meta
Record Statistics
Record Views
68
Version History
[v1] (Original Submission)
Mar 6, 2023
Verified by curator on
Mar 6, 2023
This Version Number
v1
Citations
Most Recent
This Version
URL Here
https://psecommunity.org/LAPSE:2023.17462
Original Submitter
Auto Uploader for LAPSE
Links to Related Works