LAPSE:2023.17116
Published Article
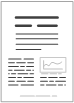
LAPSE:2023.17116
Well-Logging Prediction Based on Hybrid Neural Network Model
March 6, 2023
Well-logging is an important formation characterization and resource evaluation method in oil and gas exploration and development. However, there has been a shortage of well-logging data because Well-logging can only be measured by expensive and time-consuming field tests. In this study, we aimed to find effective machine learning techniques for well-logging data prediction, considering the temporal and spatial characteristics of well-logging data. To achieve this goal, the convolutional neural network (CNN) and the long short-term memory (LSTM) neural networks were combined to extract the spatial and temporal features of well-logging data, and the particle swarm optimization (PSO) algorithm was used to determine hyperparameters of the optimal CNN-LSTM architecture to predict logging curves in this study. We applied the proposed CNN-LSTM-PSO model, along with support vector regression, gradient-boosting regression, CNN-PSO, and LSTM-PSO models, to forecast photoelectric effect (PE) logs from other logs of the target well, and from logs of adjacent wells. Among the applied algorithms, the proposed CNN-LSTM-PSO model generated the best prediction of PE logs because it fully considers the spatio-temporal information of other well-logging curves. The prediction accuracy of the PE log using logs of the adjacent wells was not as good as that using the other well-logging data of the target well itself, due to geological uncertainties between the target well and adjacent wells. The results also show that the prediction accuracy of the models can be significantly improved with the PSO algorithm. The proposed CNN-LSTM-PSO model was found to enable reliable and efficient Well-logging prediction for existing and new drilled wells; further, as the reservoir complexity increases, the proxy model should be able to reduce the optimization time dramatically.
Record ID
Keywords
convolutional neural network, deep learning, hybrid model, long short-term memory, Particle Swarm Optimization, well-logging
Suggested Citation
Wu L, Dong Z, Li W, Jing C, Qu B. Well-Logging Prediction Based on Hybrid Neural Network Model. (2023). LAPSE:2023.17116
Author Affiliations
Wu L: Petroleum Engineering Department, Xi’an Shiyou University, Xi’an 710065, China [ORCID]
Dong Z: Petroleum Engineering Department, Xi’an Shiyou University, Xi’an 710065, China
Li W: Petroleum Engineering Department, Xi’an Shiyou University, Xi’an 710065, China
Jing C: Petroleum Engineering Department, Xi’an Shiyou University, Xi’an 710065, China
Qu B: Petroleum Engineering Department, Xi’an Shiyou University, Xi’an 710065, China
Dong Z: Petroleum Engineering Department, Xi’an Shiyou University, Xi’an 710065, China
Li W: Petroleum Engineering Department, Xi’an Shiyou University, Xi’an 710065, China
Jing C: Petroleum Engineering Department, Xi’an Shiyou University, Xi’an 710065, China
Qu B: Petroleum Engineering Department, Xi’an Shiyou University, Xi’an 710065, China
Journal Name
Energies
Volume
14
Issue
24
First Page
8583
Year
2021
Publication Date
2021-12-20
Published Version
ISSN
1996-1073
Version Comments
Original Submission
Other Meta
PII: en14248583, Publication Type: Journal Article
Record Map
Published Article
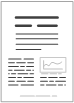
LAPSE:2023.17116
This Record
External Link
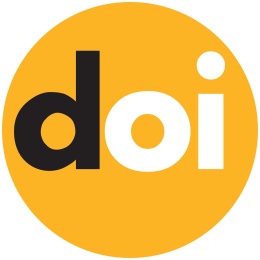
doi:10.3390/en14248583
Publisher Version
Download
Meta
Record Statistics
Record Views
82
Version History
[v1] (Original Submission)
Mar 6, 2023
Verified by curator on
Mar 6, 2023
This Version Number
v1
Citations
Most Recent
This Version
URL Here
https://psecommunity.org/LAPSE:2023.17116
Original Submitter
Auto Uploader for LAPSE
Links to Related Works