LAPSE:2023.11888
Published Article
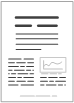
LAPSE:2023.11888
A Review of Proxy Modeling Highlighting Applications for Reservoir Engineering
February 28, 2023
Numerical models can be used for many purposes in oil and gas engineering, such as production optimization and forecasting, uncertainty analysis, history matching, and risk assessment. However, subsurface problems are complex and non-linear, and making reliable decisions in reservoir management requires substantial computational effort. Proxy models have gained much attention in recent years. They are advanced non-linear interpolation tables that can approximate complex models and alleviate computational effort. Proxy models are constructed by running high-fidelity models to gather the necessary data to create the proxy model. Once constructed, they can be a great choice for different tasks such as uncertainty analysis, optimization, forecasting, etc. The application of proxy modeling in oil and gas has had an increasing trend in recent years, and there is no consensus rule on the correct choice of proxy model. As a result, it is crucial to better understand the advantages and disadvantages of various proxy models. The existing work in the literature does not comprehensively cover all proxy model types, and there is a considerable requirement for fulfilling the existing gaps in summarizing the classification techniques with their applications. We propose a novel categorization method covering all proxy model types. This review paper provides a more comprehensive guideline on comparing and developing a proxy model compared to the existing literature. Furthermore, we point out the advantages of smart proxy models (SPM) compared to traditional proxy models (TPM) and suggest how we may further improve SPM accuracy where the literature is limited. This review paper first introduces proxy models and shows how they are classified in the literature. Then, it explains that the current classifications cannot cover all types of proxy models and proposes a novel categorization based on various development strategies. This new categorization includes four groups multi-fidelity models (MFM), reduced-order models (ROM), TPM, and SPM. MFMs are constructed based on simplifying physics assumptions (e.g., coarser discretization), and ROMs are based on dimensional reduction (i.e., neglecting irrelevant parameters). Developing these two models requires an in-depth knowledge of the problem. In contrast, TPMs and novel SPMs require less effort. In other words, they do not solve the complex underlying mathematical equations of the problem; instead, they decouple the mathematical equations into a numeric dataset and train statistical/AI-driven models on the dataset. Nevertheless, SPMs implement feature engineering techniques (i.e., generating new parameters) for its development and can capture the complexities within the reservoir, such as the constraints and characteristics of the grids. The newly introduced parameters can help find the hidden patterns within the parameters, which eventually increase the accuracy of SPMs compared to the TPMs. This review highlights the superiority of SPM over traditional statistical/AI-based proxy models. Finally, the application of various proxy models in the oil and gas industry, especially in subsurface modeling with a set of real examples, is presented. The introduced guideline in this review aids the researchers in obtaining valuable information on the current state of PM problems in the oil and gas industry.
Record ID
Keywords
application, Machine Learning, multi-fidelity, proxy model, reduced-order, sampling, sensitivity analysis, smart proxy, Surrogate Model, traditional proxy
Subject
Suggested Citation
Bahrami P, Sahari Moghaddam F, James LA. A Review of Proxy Modeling Highlighting Applications for Reservoir Engineering. (2023). LAPSE:2023.11888
Author Affiliations
Bahrami P: Department of Process Engineering, Faculty of Engineering and Applied Science, Memorial University of Newfoundland, St. John’s, NL A1C 5S7, Canada
Sahari Moghaddam F: Department of Process Engineering, Faculty of Engineering and Applied Science, Memorial University of Newfoundland, St. John’s, NL A1C 5S7, Canada
James LA: Department of Process Engineering, Faculty of Engineering and Applied Science, Memorial University of Newfoundland, St. John’s, NL A1C 5S7, Canada [ORCID]
Sahari Moghaddam F: Department of Process Engineering, Faculty of Engineering and Applied Science, Memorial University of Newfoundland, St. John’s, NL A1C 5S7, Canada
James LA: Department of Process Engineering, Faculty of Engineering and Applied Science, Memorial University of Newfoundland, St. John’s, NL A1C 5S7, Canada [ORCID]
Journal Name
Energies
Volume
15
Issue
14
First Page
5247
Year
2022
Publication Date
2022-07-20
Published Version
ISSN
1996-1073
Version Comments
Original Submission
Other Meta
PII: en15145247, Publication Type: Review
Record Map
Published Article
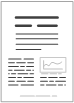
LAPSE:2023.11888
This Record
External Link
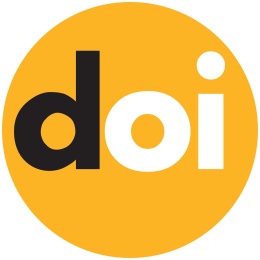
doi:10.3390/en15145247
Publisher Version
Download
Meta
Record Statistics
Record Views
112
Version History
[v1] (Original Submission)
Feb 28, 2023
Verified by curator on
Feb 28, 2023
This Version Number
v1
Citations
Most Recent
This Version
URL Here
https://psecommunity.org/LAPSE:2023.11888
Original Submitter
Auto Uploader for LAPSE
Links to Related Works