LAPSE:2023.0098
Published Article
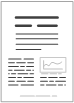
LAPSE:2023.0098
Enhance the Injection Molding Quality Prediction with Artificial Intelligence to Reach Zero-Defect Manufacturing
February 17, 2023
With the spread of the Industry 4.0 concept, implementing Artificial Intelligence approaches on the shop floor that allow companies to increase their competitiveness in the market is starting to be prioritized. Due to the complexity of the processes used in the industry, the inclusion of a real-time Quality Prediction methodology avoids a considerable number of costs to companies. This paper exposes the whole process of introducing Artificial Intelligence in plastic injection molding processes in a company in Portugal. All the implementations and methodologies used are presented, from data collection to real-time classification, such as Data Augmentation and Human-in-the-Loop labeling, among others. This approach also allows predicting and alerting with regard to process quality loss. This leads to a reduction in the production of non-compliant parts, which increases productivity and reduces costs and environmental footprint. In order to understand the applicability of this system, it was tested in different injection molding processes (traditional and stretch and blow) and with different materials and products. The results of this document show that, with the approach developed and presented, it was possible to achieve an increase in Overall Equipment Effectiveness (OEE) of up to 12%, a reduction in the process downtime of up to 9% and a significant reduction in the number of non-conforming parts produced. This improvement in key performance indicators proves the potential of this solution.
Record ID
Keywords
Artificial Intelligence, Data Augmentation, Human-in-the-Loop labeling, injection molding, OEE, predictive quality
Subject
Suggested Citation
Silva B, Marques R, Faustino D, Ilheu P, Santos T, Sousa J, Rocha AD. Enhance the Injection Molding Quality Prediction with Artificial Intelligence to Reach Zero-Defect Manufacturing. (2023). LAPSE:2023.0098
Author Affiliations
Silva B: School of Technology and Management, Polytechnic of Leiria, 2411-901 Leiria, Portugal; Muvu Technologies, 1050-052 Lisboa, Portugal; Institut de Robòtica i Informàtica Industrial, Universitat Politècnica de Catalunya BarcelonaTech (UPC), 08034 Barcelon [ORCID]
Marques R: Vipex, 2430-153 Marinha Grande, Portugal
Faustino D: Muvu Technologies, 1050-052 Lisboa, Portugal; Department of Electrical and Computer Engineering, NOVA School of Science and Technology, NOVA University of Lisbon, 2829-516 Caparica, Portugal
Ilheu P: Muvu Technologies, 1050-052 Lisboa, Portugal
Santos T: Muvu Technologies, 1050-052 Lisboa, Portugal
Sousa J: UNINOVA Centre of Technology and Systems (CTS), FCT Campus, Monte de Caparica, 2829-516 Caparica, Portugal; MEtRICs Research Center, School of Engineering, University of Minho, 4800-058 Guimarães, Portugal
Rocha AD: Department of Electrical and Computer Engineering, NOVA School of Science and Technology, NOVA University of Lisbon, 2829-516 Caparica, Portugal; UNINOVA Centre of Technology and Systems (CTS), FCT Campus, Monte de Caparica, 2829-516 Caparica, Portugal [ORCID]
Marques R: Vipex, 2430-153 Marinha Grande, Portugal
Faustino D: Muvu Technologies, 1050-052 Lisboa, Portugal; Department of Electrical and Computer Engineering, NOVA School of Science and Technology, NOVA University of Lisbon, 2829-516 Caparica, Portugal
Ilheu P: Muvu Technologies, 1050-052 Lisboa, Portugal
Santos T: Muvu Technologies, 1050-052 Lisboa, Portugal
Sousa J: UNINOVA Centre of Technology and Systems (CTS), FCT Campus, Monte de Caparica, 2829-516 Caparica, Portugal; MEtRICs Research Center, School of Engineering, University of Minho, 4800-058 Guimarães, Portugal
Rocha AD: Department of Electrical and Computer Engineering, NOVA School of Science and Technology, NOVA University of Lisbon, 2829-516 Caparica, Portugal; UNINOVA Centre of Technology and Systems (CTS), FCT Campus, Monte de Caparica, 2829-516 Caparica, Portugal [ORCID]
Journal Name
Processes
Volume
11
Issue
1
First Page
62
Year
2022
Publication Date
2022-12-27
Published Version
ISSN
2227-9717
Version Comments
Original Submission
Other Meta
PII: pr11010062, Publication Type: Journal Article
Record Map
Published Article
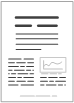
LAPSE:2023.0098
This Record
External Link
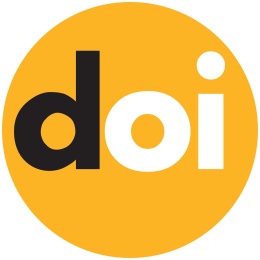
doi:10.3390/pr11010062
Publisher Version
Download
Meta
Record Statistics
Record Views
86
Version History
[v1] (Original Submission)
Feb 17, 2023
Verified by curator on
Feb 17, 2023
This Version Number
v1
Citations
Most Recent
This Version
URL Here
https://psecommunity.org/LAPSE:2023.0098
Original Submitter
Auto Uploader for LAPSE
Links to Related Works