LAPSE:2021.0070
Published Article
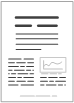
LAPSE:2021.0070
Temporal-Spatial Neighborhood Enhanced Sparse Autoencoder for Nonlinear Dynamic Process Monitoring
February 22, 2021
Data-based process monitoring methods have received tremendous attention in recent years, and modern industrial process data often exhibit dynamic and nonlinear characteristics. Traditional autoencoders, such as stacked denoising autoencoders (SDAEs), have excellent nonlinear feature extraction capabilities, but they ignore the dynamic correlation between sample data. Feature extraction based on manifold learning using spatial or temporal neighbors has been widely used in dynamic process monitoring in recent years, but most of them use linear features and do not take into account the complex nonlinearities of industrial processes. Therefore, a fault detection scheme based on temporal-spatial neighborhood enhanced sparse autoencoder is proposed in this paper. Firstly, it selects the temporal neighborhood and spatial neighborhood of the sample at the current time within the time window with a certain length, the spatial similarity and time serial correlation are used for weighted reconstruction, and the reconstruction combines the current sample as the input of the sparse stack autoencoder (SSAE) to extract the correlation features between the current sample and the neighborhood information. Two statistics are constructed for fault detection. Considering that both types of neighborhood information contain spatial-temporal structural features, Bayesian fusion strategy is used to integrate the two parts of the detection results. Finally, the superiority of the method in this paper is illustrated by a numerical example and the Tennessee Eastman process.
Record ID
Keywords
Bayesian, dynamic process, Fault Detection, sparse autoencoder, temporal-spatial neighborhood
Subject
Suggested Citation
Li N, Shi H, Song B, Tao Y. Temporal-Spatial Neighborhood Enhanced Sparse Autoencoder for Nonlinear Dynamic Process Monitoring. (2021). LAPSE:2021.0070
Author Affiliations
Li N: Key Laboratory of Advanced Control and Optimization for Chemical Processes, East China University of Science and Technology, Ministry of Education, Shanghai 200237, China
Shi H: Key Laboratory of Advanced Control and Optimization for Chemical Processes, East China University of Science and Technology, Ministry of Education, Shanghai 200237, China
Song B: Key Laboratory of Advanced Control and Optimization for Chemical Processes, East China University of Science and Technology, Ministry of Education, Shanghai 200237, China
Tao Y: Key Laboratory of Advanced Control and Optimization for Chemical Processes, East China University of Science and Technology, Ministry of Education, Shanghai 200237, China
Shi H: Key Laboratory of Advanced Control and Optimization for Chemical Processes, East China University of Science and Technology, Ministry of Education, Shanghai 200237, China
Song B: Key Laboratory of Advanced Control and Optimization for Chemical Processes, East China University of Science and Technology, Ministry of Education, Shanghai 200237, China
Tao Y: Key Laboratory of Advanced Control and Optimization for Chemical Processes, East China University of Science and Technology, Ministry of Education, Shanghai 200237, China
Journal Name
Processes
Volume
8
Issue
9
Article Number
E1079
Year
2020
Publication Date
2020-09-01
Published Version
ISSN
2227-9717
Version Comments
Original Submission
Other Meta
PII: pr8091079, Publication Type: Journal Article
Record Map
Published Article
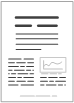
LAPSE:2021.0070
This Record
External Link
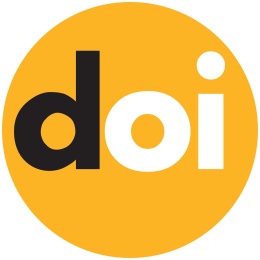
doi:10.3390/pr8091079
Publisher Version
Download
Meta
Record Statistics
Record Views
417
Version History
[v1] (Original Submission)
Feb 22, 2021
Verified by curator on
Feb 22, 2021
This Version Number
v1
Citations
Most Recent
This Version
URL Here
https://psecommunity.org/LAPSE:2021.0070
Original Submitter
Calvin Tsay
Links to Related Works