LAPSE:2020.0706
Published Article
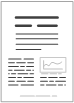
LAPSE:2020.0706
A New Scheme to Improve the Performance of Artificial Intelligence Techniques for Estimating Total Organic Carbon from Well Logs
June 23, 2020
Total organic carbon (TOC), a critical geochemical parameter of organic shale reservoirs, can be used to evaluate the hydrocarbon potential of source rocks. However, getting TOC through core analysis of geochemical experiments is costly and time-consuming. Therefore, in this paper, a TOC prediction model was built by combining the data from a case study in the Ordos Basin, China and core analysis with artificial intelligence techniques. In the study, the data of samples were optimized based on annealing algorithm (SA) and genetic algorithm (GA), named SAGA-FCM method. Then, back propagation algorithm (BPNN), least square support vector machine (LSSVM), and least square support vector machine based on particle swarm optimization algorithm (PSO-LSSVM) were built based on the data from optimization. The results show that the intelligence model constructed based on core samples data after optimization has much better performance in both training and validation accuracy than the model constructed based on original data. In addition, R² and MRSE in PSO-LSSVM are 0.9451 and 1.1883, respectively, which proves that models established with optimal dataset of core samples have higher accuracy. This study shows that the quality of sample data affects the prediction of the intelligence model dramatically and the PSO-LSSVM model can present the relationship between well log data and TOC; thus, PSO-LSSVM is a powerful tool to estimate TOC.
Record ID
Keywords
artificial intelligence techniques, geophysical logs, least square support vector machine (LSSVM), organic shale, particle swarm optimization (PSO), total organic carbon (TOC)
Subject
Suggested Citation
Wang P, Peng S. A New Scheme to Improve the Performance of Artificial Intelligence Techniques for Estimating Total Organic Carbon from Well Logs. (2020). LAPSE:2020.0706
Author Affiliations
Wang P: State Key Laboratory of Coal Resources and Safe Mining, China University of Mining & Technology (Beijing), Beijing 100083, China; College of Geoscience and Surveying Engineering, China University of Mining & Technology (Beijing), Beijing 100083, China [ORCID]
Peng S: State Key Laboratory of Coal Resources and Safe Mining, China University of Mining & Technology (Beijing), Beijing 100083, China; College of Geoscience and Surveying Engineering, China University of Mining & Technology (Beijing), Beijing 100083, China
[Login] to see author email addresses.
Peng S: State Key Laboratory of Coal Resources and Safe Mining, China University of Mining & Technology (Beijing), Beijing 100083, China; College of Geoscience and Surveying Engineering, China University of Mining & Technology (Beijing), Beijing 100083, China
[Login] to see author email addresses.
Journal Name
Energies
Volume
11
Issue
4
Article Number
E747
Year
2018
Publication Date
2018-03-26
Published Version
ISSN
1996-1073
Version Comments
Original Submission
Other Meta
PII: en11040747, Publication Type: Journal Article
Record Map
Published Article
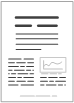
LAPSE:2020.0706
This Record
External Link
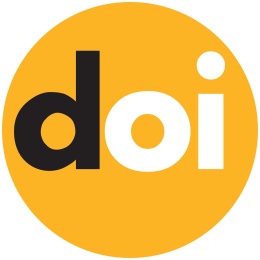
doi:10.3390/en11040747
Publisher Version
Download
Meta
Record Statistics
Record Views
409
Version History
[v1] (Original Submission)
Jun 23, 2020
Verified by curator on
Jun 23, 2020
This Version Number
v1
Citations
Most Recent
This Version
URL Here
https://psecommunity.org/LAPSE:2020.0706
Original Submitter
Calvin Tsay
Links to Related Works