LAPSE:2018.0497
Published Article
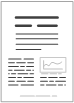
LAPSE:2018.0497
Multi-Step Ahead Wind Power Generation Prediction Based on Hybrid Machine Learning Techniques
September 21, 2018
Accurate generation prediction at multiple time-steps is of paramount importance for reliable and economical operation of wind farms. This study proposed a novel algorithmic solution using various forms of machine learning techniques in a hybrid manner, including phase space reconstruction (PSR), input variable selection (IVS), K-means clustering and adaptive neuro-fuzzy inference system (ANFIS). The PSR technique transforms the historical time series into a set of phase-space variables combining with the numerical weather prediction (NWP) data to prepare candidate inputs. A minimal redundancy maximal relevance (mRMR) criterion based filtering approach is used to automatically select the optimal input variables for the multi-step ahead prediction. Then, the input instances are divided into a set of subsets using the K-means clustering to train the ANFIS. The ANFIS parameters are further optimized to improve the prediction performance by the use of particle swarm optimization (PSO) algorithm. The proposed solution is extensively evaluated through case studies of two realistic wind farms and the numerical results clearly confirm its effectiveness and improved prediction accuracy compared to benchmark solutions.
Record ID
Keywords
input variable selection, K-means clustering, multi-step ahead prediction, neuro-fuzzy inference system, phase space reconstruction, wind power prediction
Subject
Suggested Citation
Dong W, Yang Q, Fang X. Multi-Step Ahead Wind Power Generation Prediction Based on Hybrid Machine Learning Techniques. (2018). LAPSE:2018.0497
Author Affiliations
Dong W: College of Electrical Engineering, Zhejiang University, Hangzhou 310027, China
Yang Q: College of Electrical Engineering, Zhejiang University, Hangzhou 310027, China; Jiangsu Key Construction Laboratory of IoT Application Technology, Taihu University of Wuxi, Wuxi 214064, China [ORCID]
Fang X: College of Electrical Engineering, Zhejiang University, Hangzhou 310027, China; Power China Hua Dong Engineering Corporation Limited, Hangzhou 311122, China
[Login] to see author email addresses.
Yang Q: College of Electrical Engineering, Zhejiang University, Hangzhou 310027, China; Jiangsu Key Construction Laboratory of IoT Application Technology, Taihu University of Wuxi, Wuxi 214064, China [ORCID]
Fang X: College of Electrical Engineering, Zhejiang University, Hangzhou 310027, China; Power China Hua Dong Engineering Corporation Limited, Hangzhou 311122, China
[Login] to see author email addresses.
Journal Name
Energies
Volume
11
Issue
8
Article Number
E1975
Year
2018
Publication Date
2018-07-30
Published Version
ISSN
1996-1073
Version Comments
Original Submission
Other Meta
PII: en11081975, Publication Type: Journal Article
Record Map
Published Article
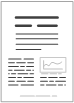
LAPSE:2018.0497
This Record
External Link
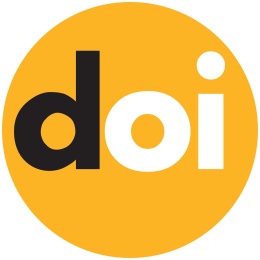
doi:10.3390/en11081975
Publisher Version
Download
Meta
Record Statistics
Record Views
599
Version History
[v1] (Original Submission)
Sep 21, 2018
Verified by curator on
Sep 21, 2018
This Version Number
v1
Citations
Most Recent
This Version
URL Here
https://psecommunity.org/LAPSE:2018.0497
Original Submitter
Calvin Tsay
Links to Related Works