LAPSE:2020.0233
Published Article
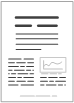
LAPSE:2020.0233
Short-Term Wind Power Prediction Based on Improved Grey Wolf Optimization Algorithm for Extreme Learning Machine
February 12, 2020
In order to improve the accuracy of wind power prediction and ensure the effective utilization of wind energy, a short-term wind power prediction model based on variational mode decomposition (VMD) and an extreme learning machine (ELM) optimized by an improved grey wolf optimization (GWO) algorithm is proposed. The original wind power sequence is decomposed into series of modal components with different center frequencies by the VMD method and some new sequences are obtained by phase space reconstruction (PSR). Then, the ELM model is established for different new time series, and the improved GWO algorithm is used to optimize its parameters. Finally, the output results are weighted and merged as the final predicted value of wind power. The root-mean-square error (RMSE), mean absolute error (MAE), and mean absolute percentage error (MAPE) of the proposed VMD-improved GWO-ELM prediction model in the paper are 5.9113%, 4.6219%, and 13.01% respectively, which are better than these of ELM, back propagation (BP), and the improved GWO-ELM model. The simulation results show that the proposed model has higher prediction accuracy than other models in short-term wind power prediction.
Record ID
Keywords
extreme learning machine, improved grey wolf optimization algorithm, phase space reconstruction, variational mode decomposition
Subject
Suggested Citation
Ding J, Chen G, Yuan K. Short-Term Wind Power Prediction Based on Improved Grey Wolf Optimization Algorithm for Extreme Learning Machine. (2020). LAPSE:2020.0233
Author Affiliations
Ding J: School of Electrical Engineering, Shanghai DianJi University, Shanghai 201306, China
Chen G: School of Electrical Engineering, Shanghai DianJi University, Shanghai 201306, China
Yuan K: School of Electrical Engineering, Shanghai DianJi University, Shanghai 201306, China
Chen G: School of Electrical Engineering, Shanghai DianJi University, Shanghai 201306, China
Yuan K: School of Electrical Engineering, Shanghai DianJi University, Shanghai 201306, China
Journal Name
Processes
Volume
8
Issue
1
Article Number
E109
Year
2020
Publication Date
2020-01-15
Published Version
ISSN
2227-9717
Version Comments
Original Submission
Other Meta
PII: pr8010109, Publication Type: Journal Article
Record Map
Published Article
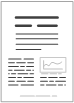
LAPSE:2020.0233
This Record
External Link
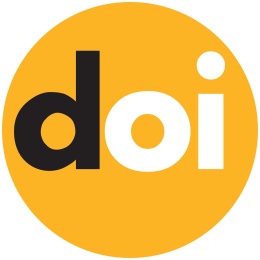
doi:10.3390/pr8010109
Publisher Version
Download
Meta
Record Statistics
Record Views
465
Version History
[v1] (Original Submission)
Feb 12, 2020
Verified by curator on
Feb 12, 2020
This Version Number
v1
Citations
Most Recent
This Version
URL Here
https://psecommunity.org/LAPSE:2020.0233
Original Submitter
Calvin Tsay
Links to Related Works