LAPSE:2019.1509
Published Article
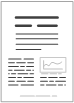
LAPSE:2019.1509
Statistical Feature Extraction for Fault Locations in Nonintrusive Fault Detection of Low Voltage Distribution Systems
December 10, 2019
This paper proposes statistical feature extraction methods combined with artificial intelligence (AI) approaches for fault locations in non-intrusive single-line-to-ground fault (SLGF) detection of low voltage distribution systems. The input features of the AI algorithms are extracted using statistical moment transformation for reducing the dimensions of the power signature inputs measured by using non-intrusive fault monitoring (NIFM) techniques. The data required to develop the network are generated by simulating SLGF using the Electromagnetic Transient Program (EMTP) in a test system. To enhance the identification accuracy, these features after normalization are given to AI algorithms for presenting and evaluating in this paper. Different AI techniques are then utilized to compare which identification algorithms are suitable to diagnose the SLGF for various power signatures in a NIFM system. The simulation results show that the proposed method is effective and can identify the fault locations by using non-intrusive monitoring techniques for low voltage distribution systems.
Record ID
Keywords
artificial intelligence (AI), distribution systems, feature extraction, non-intrusive fault monitoring (NIFM), wavelet transform
Subject
Suggested Citation
Chang HH, Linh NV. Statistical Feature Extraction for Fault Locations in Nonintrusive Fault Detection of Low Voltage Distribution Systems. (2019). LAPSE:2019.1509
Author Affiliations
Journal Name
Energies
Volume
10
Issue
5
Article Number
E611
Year
2017
Publication Date
2017-04-29
Published Version
ISSN
1996-1073
Version Comments
Original Submission
Other Meta
PII: en10050611, Publication Type: Journal Article
Record Map
Published Article
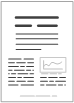
LAPSE:2019.1509
This Record
External Link
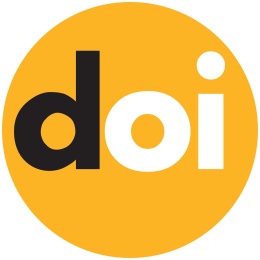
doi:10.3390/en10050611
Publisher Version
Download
Meta
Record Statistics
Record Views
442
Version History
[v1] (Original Submission)
Dec 10, 2019
Verified by curator on
Dec 10, 2019
This Version Number
v1
Citations
Most Recent
This Version
URL Here
https://psecommunity.org/LAPSE:2019.1509
Original Submitter
Calvin Tsay
Links to Related Works