LAPSE:2018.0851
Published Article
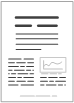
LAPSE:2018.0851
Joint Estimation of the Electric Vehicle Power Battery State of Charge Based on the Least Squares Method and the Kalman Filter Algorithm
November 16, 2018
An estimation of the power battery state of charge (SOC) is related to the energy management, the battery cycle life and the use cost of electric vehicles. When a lithium-ion power battery is used in an electric vehicle, the SOC displays a very strong time-dependent nonlinearity under the influence of random factors, such as the working conditions and the environment. Hence, research on estimating the SOC of a power battery for an electric vehicle is of great theoretical significance and application value. In this paper, according to the dynamic response of the power battery terminal voltage during a discharging process, the second-order RC circuit is first used as the equivalent model of the power battery. Subsequently, on the basis of this model, the least squares method (LS) with a forgetting factor and the adaptive unscented Kalman filter (AUKF) algorithm are used jointly in the estimation of the power battery SOC. Simulation experiments show that the joint estimation algorithm proposed in this paper has higher precision and convergence of the initial value error than a single AUKF algorithm.
Record ID
Keywords
AUKF, joint estimation, least square method with a forgetting factor
Subject
Suggested Citation
Guo X, Kang L, Yao Y, Huang Z, Li W. Joint Estimation of the Electric Vehicle Power Battery State of Charge Based on the Least Squares Method and the Kalman Filter Algorithm. (2018). LAPSE:2018.0851
Author Affiliations
Guo X: New Energy Research Center of Electric Power College, South China University of Technology, Guangzhou 510640, China; Guangdong Key Laboratory of Clean Energy Technology, South China University of Technology, Guangzhou 510640, China; College of Electrical
Kang L: New Energy Research Center of Electric Power College, South China University of Technology, Guangzhou 510640, China; Guangdong Key Laboratory of Clean Energy Technology, South China University of Technology, Guangzhou 510640, China
Yao Y: New Energy Research Center of Electric Power College, South China University of Technology, Guangzhou 510640, China; Guangdong Key Laboratory of Clean Energy Technology, South China University of Technology, Guangzhou 510640, China
Huang Z: New Energy Research Center of Electric Power College, South China University of Technology, Guangzhou 510640, China; Guangdong Key Laboratory of Clean Energy Technology, South China University of Technology, Guangzhou 510640, China
Li W: New Energy Research Center of Electric Power College, South China University of Technology, Guangzhou 510640, China; Guangdong Key Laboratory of Clean Energy Technology, South China University of Technology, Guangzhou 510640, China
[Login] to see author email addresses.
Kang L: New Energy Research Center of Electric Power College, South China University of Technology, Guangzhou 510640, China; Guangdong Key Laboratory of Clean Energy Technology, South China University of Technology, Guangzhou 510640, China
Yao Y: New Energy Research Center of Electric Power College, South China University of Technology, Guangzhou 510640, China; Guangdong Key Laboratory of Clean Energy Technology, South China University of Technology, Guangzhou 510640, China
Huang Z: New Energy Research Center of Electric Power College, South China University of Technology, Guangzhou 510640, China; Guangdong Key Laboratory of Clean Energy Technology, South China University of Technology, Guangzhou 510640, China
Li W: New Energy Research Center of Electric Power College, South China University of Technology, Guangzhou 510640, China; Guangdong Key Laboratory of Clean Energy Technology, South China University of Technology, Guangzhou 510640, China
[Login] to see author email addresses.
Journal Name
Energies
Volume
9
Issue
2
Article Number
E100
Year
2016
Publication Date
2016-02-08
Published Version
ISSN
1996-1073
Version Comments
Original Submission
Other Meta
PII: en9020100, Publication Type: Journal Article
Record Map
Published Article
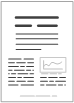
LAPSE:2018.0851
This Record
External Link
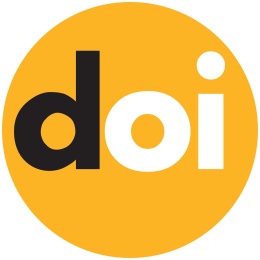
doi:10.3390/en9020100
Publisher Version
Download
Meta
Record Statistics
Record Views
637
Version History
[v1] (Original Submission)
Nov 16, 2018
Verified by curator on
Nov 16, 2018
This Version Number
v1
Citations
Most Recent
This Version
URL Here
https://psecommunity.org/LAPSE:2018.0851
Original Submitter
Calvin Tsay
Links to Related Works