LAPSE:2018.0481
Published Article
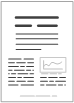
LAPSE:2018.0481
Ensemble Recurrent Neural Network Based Probabilistic Wind Speed Forecasting Approach
September 21, 2018
Wind energy is a commonly utilized renewable energy source, due to its merits of extensive distribution and rich reserves. However, as wind speed fluctuates violently and uncertainly at all times, wind power integration may affect the security and stability of power system. In this study, we propose an ensemble model for probabilistic wind speed forecasting. It consists of wavelet threshold denoising (WTD), recurrent neural network (RNN) and adaptive neuro fuzzy inference system (ANFIS). Firstly, WTD smooths the wind speed series in order to better capture its variation trend. Secondly, RNNs with different architectures are trained on the denoising datasets, operating as sub-models for point wind speed forecasting. Thirdly, ANFIS is innovatively established as the top layer of the entire ensemble model to compute the final point prediction result, in order to take full advantages of a limited number of deep-learning-based sub-models. Lastly, variances are obtained from sub-models and then prediction intervals of probabilistic forecasting can be calculated, where the variances inventively consist of modeling and forecasting uncertainties. The proposed ensemble model is established and verified on less than one-hour-ahead ultra-short-term wind speed forecasting. We compare it with other soft computing models. The results indicate the feasibility and superiority of the proposed model in both point and probabilistic wind speed forecasting.
Record ID
Keywords
adaptive neuro fuzzy inference system, deep learning, ensemble learning, probabilistic wind speed forecasting, recurrent neural network
Subject
Suggested Citation
Cheng L, Zang H, Ding T, Sun R, Wang M, Wei Z, Sun G. Ensemble Recurrent Neural Network Based Probabilistic Wind Speed Forecasting Approach. (2018). LAPSE:2018.0481
Author Affiliations
Cheng L: College of Energy and Electrical Engineering, Hohai University, Nanjing 210098, China
Zang H: College of Energy and Electrical Engineering, Hohai University, Nanjing 210098, China; Jiangsu Collaborative Innovation Center for Smart Distribution Network, Nanjing 211167, China [ORCID]
Ding T: Department of Electrical Engineering, Xi’an Jiaotong University, Xi’an 710049, China
Sun R: Electric Power Research Institute, State Grid Jiangsu Electric Power Co., Ltd., Nanjing 211103, China
Wang M: College of Energy and Electrical Engineering, Hohai University, Nanjing 210098, China
Wei Z: College of Energy and Electrical Engineering, Hohai University, Nanjing 210098, China
Sun G: College of Energy and Electrical Engineering, Hohai University, Nanjing 210098, China [ORCID]
[Login] to see author email addresses.
Zang H: College of Energy and Electrical Engineering, Hohai University, Nanjing 210098, China; Jiangsu Collaborative Innovation Center for Smart Distribution Network, Nanjing 211167, China [ORCID]
Ding T: Department of Electrical Engineering, Xi’an Jiaotong University, Xi’an 710049, China
Sun R: Electric Power Research Institute, State Grid Jiangsu Electric Power Co., Ltd., Nanjing 211103, China
Wang M: College of Energy and Electrical Engineering, Hohai University, Nanjing 210098, China
Wei Z: College of Energy and Electrical Engineering, Hohai University, Nanjing 210098, China
Sun G: College of Energy and Electrical Engineering, Hohai University, Nanjing 210098, China [ORCID]
[Login] to see author email addresses.
Journal Name
Energies
Volume
11
Issue
8
Article Number
E1958
Year
2018
Publication Date
2018-07-27
Published Version
ISSN
1996-1073
Version Comments
Original Submission
Other Meta
PII: en11081958, Publication Type: Journal Article
Record Map
Published Article
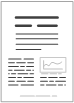
LAPSE:2018.0481
This Record
External Link
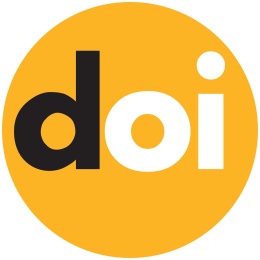
doi:10.3390/en11081958
Publisher Version
Download
Meta
Record Statistics
Record Views
613
Version History
[v1] (Original Submission)
Sep 21, 2018
Verified by curator on
Sep 21, 2018
This Version Number
v1
Citations
Most Recent
This Version
URL Here
https://psecommunity.org/LAPSE:2018.0481
Original Submitter
Calvin Tsay
Links to Related Works