LAPSE:2022.0092
Published Article
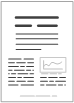
LAPSE:2022.0092
Prediction of Different Crude Oil Properties from FTIR Data with Statistical Methods, Deep and Shallow Neural Networks
October 21, 2022. Originally submitted on October 19, 2022
In recent years, Fourier Transform InfraRed (FTIR) spectrometry has been widely used to estimate different characteristics and contents of materials in many fields. Even though numerous works have been published in this area, it has still been difficult to suggest a global method that can predict the properties of crude oils from different resources based solely on FTIR data. In this study, we compare the application of several methods in order to predict particular important properties (i.e., viscosity, density, total sulfur content, total acid number, etc.) of crude oil samples from seven different Canadian oil fields. We employed chemometric methods such as Partial Least Squares regression (PLS) and principal component regression (PCR) and compared the results to the performance of neural networks (NN) with a different number of layers. These methods were evaluated by calculating the coefficient of determination (R2) and prediction root mean squared errors (RMSE). Although less complicated statistical methods like PCR and PLS could lead to excellent predictions for some properties, we found that neural networks could improve the results in other properties. In addition, the prediction accuracy of some properties, like viscosity, was improved by classification before the application of regression methods.
Record ID
Keywords
Chemometric Methods, Crude Oil Properties, Deep Neural Network, FTIR, Shallow Neural Network, Statistical Methods
Suggested Citation
Alizadeh S, Ta S, Ray AK, Samavedham L. Prediction of Different Crude Oil Properties from FTIR Data with Statistical Methods, Deep and Shallow Neural Networks. (2022). LAPSE:2022.0092
Author Affiliations
Alizadeh S*: Western University
Ta S: Western University
Ray AK: Western University
Samavedham L: National University of Singapore
* Corresponding Author
[Login] to see author email addresses.
Ta S: Western University
Ray AK: Western University
Samavedham L: National University of Singapore
* Corresponding Author
[Login] to see author email addresses.
Journal Name
CSChE Systems & Control Transactions
Volume
2
First Page
29
Last Page
30
Year
2022
Publication Date
2022-10-19
Version Comments
Revision of Version 1
Download
Meta