LAPSE:2021.0062v1
Published Article
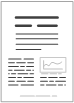
LAPSE:2021.0062v1
Machine Learning for the Classification of Alzheimer’s Disease and Its Prodromal Stage Using Brain Diffusion Tensor Imaging Data: A Systematic Review
February 22, 2021
Alzheimer’s disease is notoriously the most common cause of dementia in the elderly, affecting an increasing number of people. Although widespread, its causes and progression modalities are complex and still not fully understood. Through neuroimaging techniques, such as diffusion Magnetic Resonance (MR), more sophisticated and specific studies of the disease can be performed, offering a valuable tool for both its diagnosis and early detection. However, processing large quantities of medical images is not an easy task, and researchers have turned their attention towards machine learning, a set of computer algorithms that automatically adapt their output towards the intended goal. In this paper, a systematic review of recent machine learning applications on diffusion tensor imaging studies of Alzheimer’s disease is presented, highlighting the fundamental aspects of each work and reporting their performance score. A few examined studies also include mild cognitive impairment in the classification problem, while others combine diffusion data with other sources, like structural magnetic resonance imaging (MRI) (multimodal analysis). The findings of the retrieved works suggest a promising role for machine learning in evaluating effective classification features, like fractional anisotropy, and in possibly performing on different image modalities with higher accuracy.
Record ID
Keywords
Alzheimer’s disease, diffusion tensor imaging, Machine Learning, magnetic resonance imaging, mild cognitive impairment, support vector machine
Subject
Suggested Citation
Billeci L, Badolato A, Bachi L, Tonacci A. Machine Learning for the Classification of Alzheimer’s Disease and Its Prodromal Stage Using Brain Diffusion Tensor Imaging Data: A Systematic Review. (2021). LAPSE:2021.0062v1
Author Affiliations
Billeci L: Institute of Clinical Physiology-National Research Council of Italy (IFC-CNR), Via Moruzzi, 1, 56124 Pisa, Italy [ORCID]
Badolato A: School of Engineering, University of Pisa, Largo Lucio Lazzarino, 1, 56122 Pisa, Italy
Bachi L: Institute of Clinical Physiology-National Research Council of Italy (IFC-CNR), Via Moruzzi, 1, 56124 Pisa, Italy
Tonacci A: Institute of Clinical Physiology-National Research Council of Italy (IFC-CNR), Via Moruzzi, 1, 56124 Pisa, Italy [ORCID]
Badolato A: School of Engineering, University of Pisa, Largo Lucio Lazzarino, 1, 56122 Pisa, Italy
Bachi L: Institute of Clinical Physiology-National Research Council of Italy (IFC-CNR), Via Moruzzi, 1, 56124 Pisa, Italy
Tonacci A: Institute of Clinical Physiology-National Research Council of Italy (IFC-CNR), Via Moruzzi, 1, 56124 Pisa, Italy [ORCID]
Journal Name
Processes
Volume
8
Issue
9
Article Number
E1071
Year
2020
Publication Date
2020-09-01
Published Version
ISSN
2227-9717
Version Comments
Original Submission
Other Meta
PII: pr8091071, Publication Type: Review
Record Map
Published Article
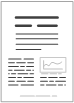
LAPSE:2021.0062v1
This Record
External Link
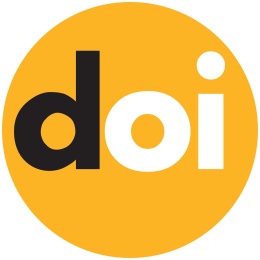
doi:10.3390/pr8091071
Publisher Version
Download
Meta
Record Statistics
Record Views
537
Version History
[v1] (Original Submission)
Feb 22, 2021
Verified by curator on
Feb 22, 2021
This Version Number
v1
Citations
Most Recent
This Version
URL Here
https://psecommunity.org/LAPSE:2021.0062v1
Original Submitter
Calvin Tsay
Links to Related Works